Read online or download Political Science eBooks for free. Keywords: politics, politicians, nation, state, political parties, parliament, democracy. Department of Political Science. Provides you free political science ebooks. Kapur, Principles of Political Science 3. To find more books about principles of political science by a c kapoor pdf.
« Previous: 2 Accumulation of Scientific KnowledgeIn Chapter 2 we present evidence that scientific research in education accumulates just as it does in the physical, life, and social sciences. Consequently, we believe that such research would be worthwhile to pursue to build further knowledge about education, and about education policy and practice. Up to this point, however, we have not addressed the questions “What constitutes scientific research?” and “Is scientific research on education different from scientific research in the social, life, and physical sciences?” We do so in this chapter.
These are daunting questions that philosophers, historians, and scientists have debated for several centuries (see Newton-Smith [2000] for a current assessment). Merton (1973), for example, saw commonality among the sciences. He described science as having four aims: universalism, the quest for general laws; organization, the quest to organize and conceptualize a set of related facts or observations; skepticism, the norm of questioning and looking for counter explanations; and communalism, the quest to develop a community that shares a set of norms or principles for doing science. In contrast, some early modern philosophers (the logical positivists) attempted to achieve unity across the sciences by reducing them all to physics, a program that ran into insuperable technical difficulties (Trant, 1991).
In short, we hold that there are both commonalities and differences across the sciences. At a general level, the sciences share a great deal in common, a set of what might be called epistemological or fundamental
principles that guide the scientific enterprise. They include seeking conceptual (theoretical) understanding, posing empirically testable and refutable hypotheses, designing studies that test and can rule out competing counterhypotheses, using observational methods linked to theory that enable other scientists to verify their accuracy, and recognizing the importance of both independent replication and generalization. It is very unlikely that any one study would possess all of these qualities. Nevertheless, what unites scientific inquiry is the primacy of empirical test of conjectures and formal hypotheses using well-codified observation methods and rigorous designs, and subjecting findings to peer review. It is, in John Dewey’s expression, “competent inquiry” that produces what philosophers call “knowledge claims” that are justified or “warranted” by pertinent, empirical evidence (or in mathematics, deductive proof). Scientific reasoning takes place amid (often quantifiable) uncertainty (Schum, 1994); its assertions are subject to challenge, replication, and revision as knowledge is refined over time. The long-term goal of much of science is to produce theory that can offer a stable encapsulation of “facts” that generalizes beyond the particular. In this chapter, then, we spell out what we see as the commonalities among all scientific endeavors.
As our work began, we attempted to distinguish scientific investigations in education from those in the social, physical, and life sciences by exploring the philosophy of science and social science; the conduct of physical, life, and social science investigations; and the conduct of scientific research on education. We also asked a panel of senior government officials who fund and manage research in education and the social and behavioral sciences, and a panel of distinguished scholars from psychometrics, linguistic anthropology, labor economics and law, to distinguish principles of evidence across fields (see National Research Council, 2001d). Ultimately, we failed to convince ourselves that at a fundamental level beyond the differences in specialized techniques and objects of inquiry across the individual sciences, a meaningful distinction could be made among social, physical, and life science research and scientific research in education. At times we thought we had an example that would demonstrate the distinction, only to find our hypothesis refuted by evidence that the distinction was not real.
Thus, the committee concluded that the set of guiding principles that apply to scientific inquiry in education are the same set of principles that
can be found across the full range of scientific inquiry. Throughout this chapter we provide examples from a variety of domains—in political science, geophysics, and education—to demonstrate this shared nature. Although there is no universally accepted description of the elements of scientific inquiry, we have found it convenient to describe the scientific process in terms of six interrelated, but not necessarily ordered,1 principles of inquiry:
Pose significant questions that can be investigated empirically.
Link research to relevant theory.
Use methods that permit direct investigation of the question.
Provide a coherent and explicit chain of reasoning.
Replicate and generalize across studies.
Disclose research to encourage professional scrutiny and critique.
We choose the phrase “guiding principles” deliberately to emphasize the vital point that they guide, but do not provide an algorithm for, scientific inquiry. Rather, the guiding principles for scientific investigations provide a framework indicating how inferences are, in general, to be supported (or refuted) by a core of interdependent processes, tools, and practices. Although any single scientific study may not fulfill all the principles—for example, an initial study in a line of inquiry will not have been replicated independently—a strong line of research is likely to do so (e.g., see Chapter 2).
We also view the guiding principles as constituting a code of conduct that includes notions of ethical behavior. In a sense, guiding principles operate like norms in a community, in this case a community of scientists; they are expectations for how scientific research will be conducted. Ideally, individual scientists internalize these norms, and the community monitors them. According to our analysis these principles of science are common to systematic study in such disciplines as astrophysics, political science, and economics, as well as to more applied fields such as medicine, agriculture, and education. The principles emphasize objectivity, rigorous thinking, open-mindedness, and honest and thorough reporting. Numerous scholars
1 | For example, inductive, deductive, and abductive modes of scientific inquiry meet these principles in different sequences. |
have commented on the common scientific “conceptual culture” that pervades most fields (see, e.g., Ziman, 2000, p. 145; Chubin and Hackett, 1990).
These principles cut across two dimensions of the scientific enterprise: the creativity, expertise, communal values, and good judgment of the people who “do” science; and generalized guiding principles for scientific inquiry. The remainder of this chapter lays out the communal values of the scientific community and the guiding principles of the process that enable well-grounded scientific investigations to flourish.
Science is a communal “form of life” (to use the expression of the philosopher Ludwig Wittgenstein [1968]), and the norms of the community take time to learn. Skilled investigators usually learn to conduct rigorous scientific investigations only after acquiring the values of the scientific community, gaining expertise in several related subfields, and mastering diverse investigative techniques through years of practice. https://spingol.netlify.app/chris-isaak-wicked-game-flac.html.
The culture of science fosters objectivity through enforcement of the rules of its “form of life”—such as the need for replicability, the unfettered flow of constructive critique, the desirability of blind refereeing—as well as through concerted efforts to train new scientists in certain habits of mind. By habits of mind, we mean things such as a dedication to the primacy of evidence, to minimizing and accounting for biases that might affect the research process, and to disciplined, creative, and open-minded thinking. These habits, together with the watchfulness of the community as a whole, result in a cadre of investigators who can engage differing perspectives and explanations in their work and consider alternative paradigms. Perhaps above all, the communally enforced norms ensure as much as is humanly possible that individual scientists—while not necessarily happy about being proven wrong—are willing to open their work to criticism, assessment, and potential revision.
Another crucial norm of the scientific “form of life,” which also depends for its efficacy on communal enforcement, is that scientists should be ethical and honest. This assertion may seem trite, even naïve. But scientific knowledge is constructed by the work of individuals, and like any other enterprise, if the people conducting the work are not open and candid, it
can easily falter. Sir Cyril Burt, a distinguished psychologist studying the heritability of intelligence, provides a case in point. He believed so strongly in his hypothesis that intelligence was highly heritable that he “doctored” data from twin studies to support his hypothesis (Tucker, 1994; Mackintosh, 1995); the scientific community reacted with horror when this transgression came to light. Examples of such unethical conduct in such fields as medical research are also well documented (see, e.g., Lock and Wells, 1996).
A different set of ethical issues also arises in the sciences that involve research with animals and humans. The involvement of living beings in the research process inevitably raises difficult ethical questions about a host of potential risks, ranging from confidentiality and privacy concerns to injury and death. Scientists must weigh the relative benefits of what might be learned against the potential risks to human research participants as they strive toward rigorous inquiry. (We consider this issue more fully in Chapters 4 and 6.)
Throughout this report we argue that science is competent inquiry that produces warranted assertions (Dewey, 1938), and ultimately develops theory that is supported by pertinent evidence. The guiding principles that follow provide a framework for how valid inferences are supported, characterize the grounds on which scientists criticize one another’s work, and with hindsight, describe what scientists do. Science is a creative enterprise, but it is disciplined by communal norms and accepted practices for appraising conclusions and how they were reached. These principles have evolved over time from lessons learned by generations of scientists and scholars of science who have continually refined their theories and methods.
SCIENTIFIC PRINCIPLE 1
Pose Significant Questions That Can Be Investigated Empirically
This principle has two parts. The first part concerns the nature of the questions posed: science proceeds by posing significant questions about the world with potentially multiple answers that lead to hypotheses or conjectures that can be tested and refuted. The second part concerns how these questions are posed: they must be posed in such a way that it is
possible to test the adequacy of alternative answers through carefully designed and implemented observations.
A crucial but typically undervalued aspect of successful scientific investigation is the quality of the question posed. Moving from hunch to conceptualization and specification of a worthwhile question is essential to scientific research. Indeed, many scientists owe their renown less to their ability to solve problems than to their capacity to select insightful questions for investigation, a capacity that is both creative and disciplined:
The formulation of a problem is often more essential than its solution, which may be merely a matter of mathematical or experimental skill. To raise new questions, new possibilities, to regard old questions from a new angle, requires creative imagination and marks real advance in science (Einstein and Infeld, 1938, p. 92, quoted in Krathwohl, 1998).
Questions are posed in an effort to fill a gap in existing knowledge or to seek new knowledge, to pursue the identification of the cause or causes of some phenomena, to describe phenomena, to solve a practical problem, or to formally test a hypothesis. A good question may reframe an older problem in light of newly available tools or techniques, methodological or theoretical. For example, political scientist Robert Putnam challenged the accepted wisdom that increased modernity led to decreased civic involvement (see Box 3-1) and his work has been challenged in turn. A question may also be a retesting of a hypothesis under new conditions or circumstances; indeed, studies that replicate earlier work are key to robust research findings that hold across settings and objects of inquiry (see Principle 5). A good question can lead to a strong test of a theory, however explicit or implicit the theory may be.
The significance of a question can be established with reference to prior research and relevant theory, as well as to its relationship with important claims pertaining to policy or practice. In this way, scientific knowledge grows as new work is added to—and integrated with—the body of material that has come before it. This body of knowledge includes theo-
BOX 3-1 In 1970 political scientist Robert Putnam was in Rome studying Italian politics when the government decided to implement a new system of regional governments throughout the country. This situation gave Putnam and his colleagues an opportunity to begin a long-term study of how government institutions develop in diverse social environments and what affects their success or failure as democratic institutions (Putnam, Leonardi, and Nanetti, 1993). Based on a conceptual framework about “institutional performance,” Putnam and his colleagues carried out three or four waves of personal interviews with government officials and local leaders, six nationwide surveys, statistical measures of institutional performance, analysis of relevant legislation from 1970 to 1984, a one-time experiment in government responsiveness, and indepth case studies in six regions from 1976 to 1989. The researchers found converging evidence of striking differences by region that had deep historical roots. The results also cast doubt on the then-prevalent view that increased modernity leads to decreased civic involvement. “The least civic areas of Italy are precisely the traditional southern villages. The civic ethos of traditional communities must not be idealized. Life in much of traditional Italy today is marked by hierarchy and exploitation, not by share-and-share alike” (p. 114). In contrast, “The most civic regions of Italy—the communities where citizens feel empowered to engage in collective deliberation about public choices and where those choices are translated most fully into effective public policies—include some of the most modern towns and cities of the peninsula. Modernization does not signal the demise of the civic community” (p. 115). The findings of Putnam and his colleagues about the relative influence of economic development and civic traditions on democratic success are less conclusive, but the weight of the evidence favors the assertion that civic tradition matters more than economic affluence. This and subsequent work on social capital (Putnam, 1995) has led to a flurry of investigations and controversy that continues today. |
ries, models, research methods (e.g., designs, measurements), and research tools (e.g., microscopes, questionnaires). Indeed, science is not only an effort to produce representations (models) of real-world phenomena by going from nature to abstract signs. Embedded in their practice, scientists also engage in the development of objects (e.g., instruments or practices); thus, scientific knowledge is a by-product of both technological activities and analytical activities (Roth, 2001). A review of theories and prior research relevant to a particular question can simply establish that it has not been answered before. Once this is established, the review can help shape alternative answers, the design and execution of a study by illuminating if and how the question and related conjectures have already been examined, as well as by identifying what is known about sampling, setting, and other important context.2
Donald Stokes’ work (Stokes, 1997) provides a useful framework for thinking about important questions that can advance scientific knowledge and method (see Figure 3-1). In Pasteur’s Quadrant, he provided evidence that the conception of research-based knowledge as moving in a linear progression from fundamental science to applied science does not reflect how science has historically advanced. He provided several examples demonstrating that, instead, many advancements in science occurred as a result of “use-inspired research,” which simultaneously draws on both basic and applied research. Stokes (1997, p. 63) cites Brooks (1967) on basic and applied work:
Work directed toward applied goals can be highly fundamental in character in that it has an important impact on the conceptual structure or outlook of a field. Moreover, the fact that research is of such a nature that it can be applied does not mean that it is not also basic.
2 | We recognize that important scientific discoveries are sometimes made when a competent observer notes a strange or interesting phenomenon for the first time. In these cases, of course, no prior literature exists to shape the investigation. And new fields and disciplines need to start somewhere. Our emphasis on linking to prior literature in this principle, then, applies generally to relatively established domains and fields. |
FIGURE 3-1. Quadrant model of scientific research.
SOURCE: Stokes (1997, p. 73). Reprinted with permission.
Stokes’ model clearly applies to research in education, where problems of practice and policy provide a rich source for important—and often highly fundamental in character—research questions.
Put simply, the term “empirical” means based on experience through the senses, which in turn is covered by the generic term observation. Since science is concerned with making sense of the world, its work is necessarily grounded in observations that can be made about it. Thus, research questions
must be posed in ways that potentially allow for empirical investigation.3 For example, both Milankovitch and Muller could collect data on the Earth’s orbit to attempt to explain the periodicity in ice ages (see Box 3-2). Likewise, Putnam could collect data from natural variations in regional government to address the question of whether modernization leads to the demise of civic community (Box 3-1), and the Tennessee state legislature could empirically assess whether reducing class size improves students’ achievement in early grades (Box 3-3) because achievement data could be collected on students in classes of varying sizes. In contrast, questions such as: “ Should all students be required to say the pledge of allegiance?” cannot be submitted to empirical investigation and thus cannot be examined scientifically. Answers to these questions lie in realms other than science.
SCIENTIFIC PRINCIPLE 2
Link Research to Relevant Theory
Scientific theories are, in essence, conceptual models that explain some phenomenon. They are “nets cast to catch what we call ‘the world’…we endeavor to make the mesh ever finer and finer” (Popper, 1959, p. 59). Indeed, much of science is fundamentally concerned with developing and testing theories, hypotheses, models, conjectures, or conceptual frameworks that can explain aspects of the physical and social world. Examples of well-known scientific theories include evolution, quantum theory, and the theory of relativity.
In the social sciences and in education, such “grand” theories are rare. To be sure, generalized theoretical understanding is still a goal. However, some research in the social sciences seeks to achieve deep understanding of particular events or circumstances rather than theoretical understanding that will generalize across situations or events. Between these extremes lies the bulk of social science theory or models, what Merton (1973) called
3 | Philosophers of science have long debated the meaning of the term empirical. As we state here, in one sense the empirical nature of science means that assertions about the world must be warranted by, or at least constrained by, explicit observation of it. However, we recognize that in addition to direct observation, strategies like logical reasoning and mathematical analysis can also provide empirical support for scientific assertions. |
BOX 3-2 During the past 1 billion years, the earth’s climate has fluctuated between cold periods, when glaciers scoured the continents, and ice-free warm periods. Serbian mathematician Milutin Milankovitch in the 1930s posited the textbook explanation for these cycles, which was accepted as canon until recently (Milankovitch, 1941/1969; Berger, Imbrie, Hays, Kukla, and Saltzman, 1984). He based his theory on painstaking measurements of the eccentricity—or out-of-roundness—of the Earth’s orbit, which changed from almost perfectly circular to slightly oval and back every 100,000 years, matching the interval between glaciation periods. Subsequently, however, analysis of light energy absorbed by Earth, measured from the content of organic material in geological sediment cores, raised doubts about this correlation as a causal mechanism (e.g., MacDonald and Sertorio, 1990). The modest change in eccentricity did not make nearly enough difference in incident sunlight to produce the required change in thermal absorption. Another problem with Milankovitch’s explanation was that the geologic record showed some glaciation periods beginning before the orbital changes that supposedly caused them (Broecker, 1992; Winograd, Coplen, and Landwehr, 1992). Astrophysicist Richard Muller then suggested an alternative mechanism, based on a different aspect of the Earth’s orbit (Muller, 1994; Karner and Muller, 2000; also see Grossman, 2001). Muller hypothesized that it is the Earth’s orbit in and out of the ecliptic that has been responsible for Earth’s cycli |
mid-range theories that attempt to account for some aspect of the social world. Examples of such mid-range theories or explanatory models can be found in the physical and the social sciences.
These theories are representations or abstractions of some aspect of reality that one can only approximate by such models. Molecules, fields, or black holes are classic explanatory models in physics; the genetic code and the contractile filament model of muscle are two in biology. Similarly,
cal glaciation periods. He based the hypothesis on astronomical observations showing that the regions above and below the ecliptic are laden with cosmic dust, which would cool the planet. Muller’s “inclination theory” received major support when Kenneth Farley (1995) published a paper on cosmic dust in sea sediments. Farley had begun his research project in an effort to refute the Muller inclination model, but discovered—to his surprise— that cosmic dust levels did indeed wax and wane in sync with the ice ages. As an immediate cause of the temperature change, Muller proposed that dust from space would influence the cloud cover on Earth and the amount of greenhouse gases—mainly carbon dioxide—in the atmosphere. Indeed, measurements of oxygen isotopes in trapped air bubbles and other properties from a 400,000-year-long Antarctic ice core by paleoceanographer Nicholas Shackleton (2001) provided more confirming evidence. To gain greater understanding of these processes, geochronologists are seeking new “clocks” to determine more accurately the timing of events in the Earth’s history (e.g., Feng and Vasconcelos, 2001), while geochemists look for new ways of inferring temperature from composition of gasses trapped deep in ice or rock (see Pope and Giles, 2001). Still, no one knows how orbital variations would send the carbon dioxide into and out of the atmosphere. And there are likely to be other significant geologic factors besides carbon dioxide that control climate. There is much work still to be done to sort out the complex variables that are probably responsible for the ice ages. |
culture, socioeconomic status, and poverty are classical models in anthropology, sociology, and political science. In program evaluation, program developers have ideas about the mechanism by which program inputs affect targeted outcomes; evaluations translate and test these ideas through a “program theory” that guides the work (Weiss, 1998a).
Theory enters the research process in two important ways. First, scientific research may be guided by a conceptual framework, model, or theory
that suggests possible questions to ask or answers to the question posed.4 The process of posing significant questions typically occurs before a study is conducted. Researchers seek to test whether a theory holds up under certain circumstances. Here the link between question and theory is straightforward. For example, Putnam based his work on a theoretical conception of institutional performance that related civic engagement and modernization.
A research question can also devolve from a practical problem (Stokes, 1997; see discussion above). In this case, addressing a complex problem like the relationship between class size and student achievement may require several theories. Different theories may give conflicting predictions about the problem’s solution, or various theories might have to be reconciled to address the problem. Indeed, the findings from the Tennessee class size reduction study (see Box 3-3) have led to several efforts to devise theoretical understandings of how class size reduction may lead to better student achievement. Scientists are developing models to understand differences in classroom behavior between large and small classes that may ultimately explain and predict changes in achievement (Grissmer and Flannagan, 2000).
A second more subtle way that theoretical understanding factors into the research process derives from the fact that all scientific observations are “theory laden” (Kuhn, 1962). That is, the choice of what to observe and how to observe it is driven by an organizing conception—explicit or tacit— of the problem or topic. Thus, theory drives the research question, the use of methods, and the interpretation of results.
SCIENTIFIC PRINCIPLE 3
Use Methods That Permit Direct Investigation of the Question
Research methods—the design for collecting data and the measurement and analysis of variables in the design—should be selected in light of a research question, and should address it directly. Methods linked directly to problems permit the development of a logical chain of reasoning based
4 | The process of posing significant questions or hypotheses may occur, as well, at the end of a study (e.g., Agar, 1996), or over the course of an investigation as understanding of the facets of the problem evolves (e.g., Brown, 1992). |
on the interplay among investigative techniques, data, and hypotheses to reach justifiable conclusions. For clarity of discussion, we separate out the link between question and method (see Principle 3) and the rigorous reasoning from evidence to theory (see Principle 4). In the actual practice of research, such a separation cannot be achieved.
Debates about method—in many disciplines and fields—have raged for centuries as researchers have battled over the relative merit of the various techniques of their trade. The simple truth is that the method used to conduct scientific research must fit the question posed, and the investigator must competently implement the method. Particular methods are better suited to address some questions rather than others. The rare choice in the mid 1980s in Tennessee to conduct a randomized field trial, for example, enabled stronger inferences about the effects of class size reduction on student achievement (see Box 3-3) than would have been possible with other methods.
This link between question and method must be clearly explicated and justified; a researcher should indicate how a particular method will enable competent investigation of the question of interest. Moreover, a detailed description of method—measurements, data collection procedures, and data analyses—must be available to permit others to critique or replicate the study (see Principle 5). Finally, investigators should identify potential methodological limitations (such as insensitivity to potentially important variables, missing data, and potential researcher bias).
The choice of method is not always straightforward because, across all disciplines and fields, a wide range of legitimate methods—both quantitative and qualitative—are available to the researcher. For example when considering questions about the natural universe—from atoms to cells to black holes—profoundly different methods and approaches characterize each sub-field. While investigations in the natural sciences are often dependent on the use of highly sophisticated instrumentation (e.g., particle accelerators, gene sequencers, scanning tunneling microscopes), more rudimentary methods often enable significant scientific breakthroughs. For example, in 1995 two Danish zoologists identified an entirely new phylum of animals from a species of tiny rotifer-like creatures found living on the mouthparts of lobsters, using only a hand lens and light microscope (Wilson, 1998, p. 63).
BOX 3-3 Although research on the effects of class size reduction on students’ achievement dates back 100 years, Glass and Smith (1978) reported the first comprehensive statistical synthesis (meta-analysis) of the literature and concluded that, indeed, there were small improvements in achievement when class size was reduced (see also Glass, Cahen, Smith, and Filby, 1982; Bohrnstedt and Stecher, 1999). However, the Glass and Smith study was criticized (e.g., Robinson and Wittebols, 1986; Slavin, 1989) on a number of grounds, including the selection of some of the studies for the meta-analysis (e.g., tutoring, college classes, atypically small classes). Some subsequent reviews reached conclusions similar to Glass and Smith (e.g., Bohrnstedt and Stetcher, 1999; Hedges, Laine, and Greenwald, 1994; Robinson and Wittebols, 1986) while others did not find consistent evidence of a positive effect (e.g., Hanushek, 1986, 1999a; Odden, 1990; Slavin, 1989). Does reducing class size improve students’ achievement? In the midst of controversy, the Tennessee state legislature asked just this question and funded a randomized experiment to find out, an experiment that Harvard statistician Frederick Mosteller (1995, p. 113) called “. . . one of the most important educational investigations ever carried out.” A total of 11,600 elementary school students and their teachers in 79 schools across the state were randomly assigned to one of three class-size conditions: small class (13-17 students), regular class |
If a research conjecture or hypothesis can withstand scrutiny by multiple methods its credibility is enhanced greatly. As Webb, Campbell, Schwartz, and Sechrest (1966, pp. 173-174) phrased it: “When a hypothesis can survive the confrontation of a series of complementary methods of testing, it contains a degree of validity unattainable by one tested within the more constricted framework of a single method.” Putnam’s study (see Box 3-1) provides an example in which both quantitative and qualitative methods were applied in a longitudinal design (e.g., interview, survey, statistical estimate of institutional performance, analysis of legislative docu-
(22-26 students), or regular class (22-26 students) with a full-time teacher’s aide (for descriptions of the experiment, see Achilles, 1999; Finn and Achilles, 1990; Folger and Breda, 1989; Krueger, 1999; Word et al., 1990). The experiment began with a cohort of students who entered kindergarten in 1985, and lasted 4 years. After third grade, all students returned to regular size classes. Although students were supposed to stay in their original treatment conditions for four years, not all did. Some were randomly reassigned between regular and regular/aide conditions in the first grade while about 10 percent switched between conditions for other reasons (Krueger and Whitmore, 2000). Three findings from this experiment stand out. First, students in small classes outperformed students in regular size classes (with or without aides). Second, the benefits of class-size reduction were much greater for minorities (primarily African American) and inner-city children than others (see, e.g., Finn and Achilles, 1990, 1999; but see also Hanushek, 1999b). And third, even though students returned to regular classes in fourth grade, the reduced class-size effect persisted in affecting whether they took college entrance examinations and on their examination performance (Krueger and Whitmore, 2001).*
|
ments) to generate converging evidence about the effects of modernization on civic community. New theories about the periodicity of the ice ages, similarly, were informed by multiple methods (e.g., astronomical observations of cosmic dust, measurements of oxygen isotopes). The integration and interaction of multiple disciplinary perspectives—with their varying methods—often accounts for scientific progress (Wilson, 1998); this is evident, for example, in the advances in understanding early reading skills described in Chapter 2. This line of work features methods that range from neuroimaging to qualitative classroom observation.
We close our discussion of this principle by noting that in many sciences, measurement is a key aspect of research method. This is true for many research endeavors in the social sciences and education research, although not for all of them. If the concepts or variables are poorly specified or inadequately measured, even the best methods will not be able to support strong scientific inferences. The history of the natural sciences is one of remarkable development of concepts and variables, as well as the tools (instrumentation) to measure them. Measurement reliability and validity is particularly challenging in the social sciences and education (Messick, 1989). Sometimes theory is not strong enough to permit clear specification and justification of the concept or variable. Sometimes the tool (e.g., multiple-choice test) used to take the measurement seriously under-represents the construct (e.g., science achievement) to be measured. Sometimes the use of the measurement has an unintended social consequence (e.g., the effect of teaching to the test on the scope of the curriculum in schools).
And sometimes error is an inevitable part of the measurement process. In the physical sciences, many phenomena can be directly observed or have highly predictable properties; measurement error is often minimal. (However, see National Research Council [1991] for a discussion of when and how measurement in the physical sciences can be imprecise.) In sciences that involve the study of humans, it is essential to identify those aspects of measurement error that attenuate the estimation of the relationships of interest (e.g., Shavelson, Baxter, and Gao, 1993). By investigating those aspects of a social measurement that give rise to measurement error, the measurement process itself will often be improved. Regardless of field of study, scientific measurements should be accompanied by estimates of uncertainty whenever possible (see Principle 4 below).
SCIENTIFIC PRINCIPLE 4
Provide Coherent, Explicit Chain of Reasoning
The extent to which the inferences that are made in the course of scientific work are warranted depends on rigorous reasoning that systematically and logically links empirical observations with the underlying theory and the degree to which both the theory and the observations are linked to the question or problem that lies at the root of the investigation. There
is no recipe for determining how these ingredients should be combined; instead, what is required is the development of a logical “chain of reasoning” (Lesh, Lovitts, and Kelly, 2000) that moves from evidence to theory and back again. This chain of reasoning must be coherent, explicit (one that another researcher could replicate), and persuasive to a skeptical reader (so that, for example, counterhypotheses are addressed).
All rigorous research—quantitative and qualitative—embodies the same underlying logic of inference (King, Keohane, and Verba, 1994). This inferential reasoning is supported by clear statements about how the research conclusions were reached: What assumptions were made? How was evidence judged to be relevant? How were alternative explanations considered or discarded? How were the links between data and the conceptual or theoretical framework made?
The nature of this chain of reasoning will vary depending on the design of the study, which in turn will vary depending on the question that is being investigated. Will the research develop, extend, modify, or test a hypothesis? Does it aim to determine: What works? How does it work? Under what circumstances does it work? If the goal of the research is to test a hypothesis, stated in the form of an “if-then” rule, successful inference may depend on measuring the extent to which the rule predicts results under a variety of conditions. If the goal is to produce a description of a complex system, such as a subcellular organelle or a hierarchical social organization, successful inference may rather depend on issues of fidelity and internal consistency of the observational techniques applied to diverse components and the credibility of the evidence gathered. The research design and the inferential reasoning it enables must demonstrate a thorough understanding of the subtleties of the questions to be asked and the procedures used to answer them.
Muller (1994), for example, collected data on the inclination of the Earth’s orbit over a 100,000 year cycle, correlated it with the occurrence of ice ages, ruled out the plausibility of orbital eccentricity as a cause for the occurrence of ice ages, and inferred that the bounce in the Earth’s orbit likely caused the ice ages (see Box 3-2). Putnam used multiple methods to subject to rigorous testing his hypotheses about what affects the success or failure of democratic institutions as they develop in diverse social environments to rigorous testing, and found the weight of the evidence favored
the assertion that civic tradition matters more than economic affluence (see Box 3-1). And Baumeister, Bratslavsky, Muraven, and Tice (1998) compared three competing theories and used randomized experiments to conclude that a “psychic energy” hypothesis best explained the important psychological characteristic of “will power” (see “Application of the Principles”).
This principle has several features worthy of elaboration. Assumptions underlying the inferences made should be clearly stated and justified. Moreover, choice of design should both acknowledge potential biases and plan for implementation challenges.
Estimates of error must also be made. Claims to knowledge vary substantially according to the strength of the research design, theory, and control of extraneous variables and by systematically ruling out possible alternative explanations. Although scientists always reason in the presence of uncertainty, it is critical to gauge the magnitude of this uncertainty. In the physical and life sciences, quantitative estimates of the error associated with conclusions are often computed and reported. In the social sciences and education, such quantitative measures are sometimes difficult to generate; in any case, a statement about the nature and estimated magnitude of error must be made in order to signal the level of certainty with which conclusions have been drawn.
Perhaps most importantly, the reasoning about evidence should identify, consider, and incorporate, when appropriate, the alternative, competing explanations or rival “answers” to the research question. To make valid inferences, plausible counterexplanations must be dealt with in a rational, systematic, and compelling way.5 The validity—or credibility—of a hypothesis is substantially strengthened if alternative counterhypotheses can be ruled out and the favored one thereby supported. Well-known research designs (e.g., Campbell and Stanley [1963] in educational psychology; Heckman [1979, 1980a, 1980b, 2001] and Goldberger [1972, 1983] in
5 | In reporting, too, it is important to clarify that rival hypotheses are possible and that conclusions are not presented as if they were gospel. Murphy and colleagues call this “‘fair-dealing’—wariness of presenting the perspective of one group as if it defined a single truth about the phenomenon, while paying scant attention to other perspectives” (Murphy, Dingwall, Greatbatch, Parker, and Watson, 1998, p. 192). |
economics; and Rosenbaum and Rubin [1983, 1984] in statistics) have been crafted to guard researchers against specific counterhypotheses (or “threats to validity”). One example, often called “selectivity bias,” is the counterhypothesis that differential selection (not the treatment) caused the outcome—that participants in the experimental treatment systematically differed from participants in the traditional (control) condition in ways that mattered importantly to the outcome. A cell biologist, for example, might unintentionally place (select) heart cells with a slight glimmer into an experimental group and others into a control group, thus potentially biasing the comparison between the groups of cells. The potential for a biased—or unfair—comparison arises because the shiny cells could differ systematically from the others in ways that affect what is being studied.
Selection bias is a pervasive problem in the social sciences and education research. To illustrate, in studying the effects of class-size reduction, credentialed teachers are more likely to be found in wealthy school districts that have the resources to reduce class size than in poor districts. This fact raises the possibility that higher achievement will be observed in the smaller classes due to factors other than class size (e.g. teacher effects). Random assignment to “treatment” is the strongest known antidote to the problem of selection bias (see Chapter 5).
A second counterhypothesis contends that something in the research participants’ history that co-occurred with the treatment caused the outcome, not the treatment itself. For example, U.S. fourth-grade students outperformed students in others countries on the ecology subtest of the Third International Mathematics and Science Study. One (popular) explanation of this finding was that the effect was due to their schooling and the emphasis on ecology in U.S. elementary science curricula. A counter-hypothesis, one of history, posits that their high achievement was due to the prevalence of ecology in children’s television programming. A control group that has the same experiences as the experimental group except for the “treatment” under study is the best antidote for this problem.
A third prevalent class of alternative interpretations contends that an outcome was biased by the measurement used. For example, education effects are often judged by narrowly defined achievement tests that focus on factual knowledge and therefore favor direct-instruction teaching tech-
niques. Multiple achievement measures with high reliability (consistency) and validity (accuracy) help to counter potential measurement bias.
The Tennessee class-size study was designed primarily to eliminate all possible known explanations, except for reduced class size, in comparing the achievement of children in regular classrooms against achievement in reduced size classrooms. It did this. Complications remained, however. About ten percent of students moved out of their originally assigned condition (class size), weakening the design because the comparative groups did not remain intact to enable strict comparisons. However, most scholars who subsequently analyzed the data (e.g., Krueger and Whitmore, 2001), while limited by the original study design, suggested that these infidelities did not affect the main conclusions of the study that smaller class size caused slight improvements in achievement. Students in classes of 13-17 students outperformed their peers in larger classes, on average, by a small margin.
SCIENTIFIC PRINCIPLE 5
Replicate and Generalize Across Studies
Replication and generalization strengthen and clarify the limits of scientific conjectures and theories. By replication we mean, at an elementary level, that if one investigator makes a set of observations, another investigator can make a similar set of observations under the same conditions. Replication in this sense comes close to what psychometricians call reliability—consistency of measurements from one observer to another, from one task to another parallel task, from one occasion to another occasion. Estimates of these different types of reliability can vary when measuring a given construct: for example, in measuring performance of military personnel (National Research Council, 1991), multiple observers largely agreed on what they observed within tasks; however, enlistees’ performance across parallel tasks was quite inconsistent.
At a somewhat more complex level, replication means the ability to repeat an investigation in more than one setting (from one laboratory to another or from one field site to a similar field site) and reach similar conclusions. To be sure, replication in the physical sciences, especially with inanimate objects, is more easily achieved than in social science or education; put another way, the margin of error in social science replication is usually
much greater than in physical science replication. The role of contextual factors and the lack of control that characterizes work in the social realm require a more nuanced notion of replication. Nevertheless, the typically large margins of error in social science replications do not preclude their identification.
Having evidence of replication, an important goal of science is to understand the extent to which findings generalize from one object or person to another, from one setting to another, and so on. To this end, a substantial amount of statistical machinery has been built both to help ensure that what is observed in a particular study is representative of what is of larger interest (i.e., will generalize) and to provide a quantitative measure of the possible error in generalizing. Nonstatistical means of generalization (e.g., triangulation, analytic induction, comparative analysis) have also been developed and applied in genres of research, such as ethnography, to understand the extent to which findings generalize across time, space, and populations. Subsequent applications, implementations, or trials are often necessary to assure generalizability or to clarify its limits. For example, since the Tennessee experiment, additional studies of the effects of class size reduction on student learning have been launched in settings other than Tennessee to assess the extent to which the findings generalize (e.g., Hruz, 2000).
In the social sciences and education, many generalizations are limited to particular times and particular places (Cronbach, 1975). This is because the social world undergoes rapid and often significant change; social generalizations, as Cronbach put it, have a shorter “half-life” than those in the physical world. Campbell and Stanley (1963) dubbed the extent to which the treatment conditions and participant population of a study mirror the world to which generalization is desired the “external validity” of the study. Consider, again, the Tennessee class-size research; it was undertaken in a set of schools that had the desire to participate, the physical facilities to accommodate an increased number of classrooms, and adequate teaching staff. Governor Wilson of California “overgeneralized” the Tennessee study, ignoring the specific experimental conditions of will and capacity and implemented class-size reduction in more than 95 percent of grades K-3 in the state. Not surprisingly, most researchers studying California have
concluded that the Tennessee findings did not entirely generalize to a different time, place, and context (see, e.g., Stecher and Bohrnstedt, 2000).6
SCIENTIFIC PRINCIPLE 6
Disclose Research to Encourage Professional Scrutiny and Critique
We argue in Chapter 2 that a characteristic of scientific knowledge accumulation is its contested nature. Here we suggest that science is not only characterized by professional scrutiny and criticism, but also that such criticism is essential to scientific progress. Scientific studies usually are elements of a larger corpus of work; furthermore, the scientists carrying out a particular study always are part of a larger community of scholars. Reporting and reviewing research results are essential to enable wide and meaningful peer review. Results are traditionally published in a specialty journal, in books published by academic presses, or in other peer-reviewed publications. In recent years, an electronic version may accompany or even substitute for a print publication.7 Results may be debated at professional conferences. Regardless of the medium, the goals of research reporting are to communicate the findings from the investigation; to open the study to examination, criticism, review, and replication (see Principle 5) by peer investigators; and ultimately to incorporate the new knowledge into the prevailing canon of the field.8
6 | A question arises as to whether this is a failure to generalize or a problem of poor implementation. The conditions under which Tennessee implemented the experiment were not reproduced in California with the now known consequence of failure to replicate and generalize. |
7 | The committee is concerned that the quality of peer review in electronic modes of dissemination varies greatly and sometimes cannot be easily assessed from its source. While the Internet is providing new and exciting ways to connect scientists and promote scientific debate, the extent to which the principles of science are met in some electronically posted work is often unclear. |
8 | Social scientists and education researchers also commonly publish information about new knowledge for practitioners and the public. In those cases, the research must be reported in accessible ways so that readers can understand the researcher’s procedures and evaluate the evidence, interpretations, and arguments. |
The goal of communicating new knowledge is self-evident: research results must be brought into the professional and public domain if they are to be understood, debated, and eventually become known to those who could fruitfully use them. The extent to which new work can be reviewed and challenged by professional peers depends critically on accurate, comprehensive, and accessible records of data, method, and inferential reasoning. This careful accounting not only makes transparent the reasoning that led to conclusions—promoting its credibility—but it also allows the community of scientists and analysts to comprehend, to replicate, and otherwise to inform theory, research, and practice in that area.
Many nonscientists who seek guidance from the research community bemoan what can easily be perceived as bickering or as an indication of “bad” science. Quite the contrary: intellectual debate at professional meetings, through research collaborations, and in other settings provide the means by which scientific knowledge is refined and accepted; scientists strive for an “open society” where criticism and unfettered debate point the way to advancement. Through scholarly critique (see, e.g., Skocpol, 1996) and debate, for example, Putnam’s work has stimulated a series of articles, commentary, and controversy in research and policy circles about the role of “social capital” in political and other social phenomena (Winter, 2000). And the Tennessee class size study has been the subject of much scholarly debate, leading to a number of follow-on analyses and launching new work that attempts to understand the process by which classroom behavior may shift in small classes to facilitate learning. However, as Lagemann (2000) has observed, for many reasons the education research community has not been nearly as critical of itself as is the case in other fields of scientific study.
The committee considered a wide range of literature and scholarship to test its ideas about the guiding principles. We realized, for example, that empiricism, while a hallmark of science, does not uniquely define it. A poet can write from first-hand experience of the world, and in this sense is an empiricist. And making observations of the world, and reasoning about their experience, helps both literary critics and historians create the
Principles Of Political Science By A C Kapoor Ebook Reader Free
interpretive frameworks that they bring to bear in their scholarship. But empirical method in scientific inquiry has different features, like codified procedures for making observations and recognizing sources of bias associated with particular methods,9 and the data derived from these observations are used specifically as tools to support or refute knowledge claims. Finally, empiricism in science involves collective judgments based on logic, experience, and consensus.
Another hallmark of science is replication and generalization. Humanists do not seek replication, although they often attempt to create work that generalizes (say) to the “human condition.” However, they have no formal logic of generalization, unlike scientists working in some domains (e.g., statistical sampling theory). In sum, it is clear that there is no bright line that distinguishes science from nonscience or high-quality science from low-quality science. Rather, our principles can be used as general guidelines for understanding what can be considered scientific and what can be considered high-quality science (see, however, Chapters 4 and 5 for an elaboration).
To show how our principles help differentiate science from other forms of scholarship, we briefly consider two genres of education inquiry published in refereed journals and books. We do not make a judgment about the worth of either form of inquiry; although we believe strongly in the merits of scientific inquiry in education research and more generally, that “science” does not mean “good.” Rather, we use them as examples to illustrate the distinguishing character of our principles of science. The first—connoisseurship—grew out of the arts and humanities (e.g., Eisner, 1991) and does not claim to be scientific. The second—portraiture—claims to straddle the fence between humanistic and scientific inquiry (e.g., Lawrence-Lightfoot and Davis, 1997).
Eisner (1991, p. 7) built a method for education inquiry firmly rooted in the arts and humanities, arguing that “there are multiple ways in which the world can be known: Artists, writers, and dancers, as well as scientists, have important things to tell about the world.” His method of inquiry combines connoisseurship (the art of appreciation), which “aims to
9 | We do not claim that any one investigator or observational method is “objective.” Rather, the guiding principles are established to guard against bias through rigorous methods and a critical community. |
appreciate the qualities . . . that constitute an act, work, or object and, typically . . . to relate these to the contextual and antecedent conditions” (p. 85) with educational criticism (the art of disclosure), which provides “connoisseurship with a public face” (p. 85). The goal of this genre of research is to enable readers to enter an event and to participate in it. To this end, the educational critic—through educational connoisseurship— must capture the key qualities of the material, situation, and experience and express them in text (“criticism”) to make what the critic sees clear to others. “To know what schools are like, their strengths and their weaknesses, we need to be able to see what occurs in them, and we need to be able to tell others what we have seen in ways that are vivid and insightful” (Eisner, 1991, p. 23, italics in original).
The grounds for his knowledge claims are not those in our guiding principles. Rather, credibility is established by: (1) structural corroboration—“multiple types of data are related to each other” (p. 110) and “disconfirming evidence and contradictory interpretations” (p. 111; italics in original) are considered; (2) consensual validation—“agreement among competent others that the description, interpretation, evaluation, and thematics of an educational situation are right” (p. 112); and (3) referential adequacy— “the extent to which a reader is able to locate in its subject matter the qualities the critic addresses and the meanings he or she ascribes to these” (p. 114). While sharing some features of our guiding principles (e.g., ruling out counterinterpretations to the favored interpretation), this humanistic approach to knowledge claims builds on a very different epistemology; the key scientific concepts of reliability, replication, and generalization, for example, are quite different. We agree with Eisner that such approaches fall outside the purview of science and conclude that our guiding principles readily distinguish them.
Portraiture (Lawrence-Lightfoot, 1994; Lawrence-Lightfoot and Davis, 1997) is a qualitative research method that aims to “record and interpret the perspectives and experience of the people they [the researchers] are studying, documenting their [the research participants’] voices and their visions—their authority, knowledge, and wisdom” (Lawrence-Lightfoot and Davis, 1997, p. xv). In contrast to connoisseurship’s humanist orientation, portraiture “seeks to join science and art” (Lawrence-Lightfoot and Davis, 1997, p. xv) by “embracing the intersection of aesthetics and empiricism” (p. 6). The standard for judging the quality of portraiture is authenticity,
“. . . capturing the essence and resonance of the actors’ experience and perspective through the details of action and thought revealed in context” (p. 12). When empirical and literary themes come together (called “resonance”) for the researcher, the actors, and the audience, “we speak of the portrait as achieving authenticity” (p. 260).
In I’ve Known Rivers, Lawrence-Lightfoot (1994) explored the life stories of six men and women:
. . . using the intensive, probing method of ‘human archeology’—a name I [Lawrence-Lightfoot] coined for this genre of portraiture as a way of trying to convey the depth and penetration of the inquiry, the richness of the layers of human experience, the search for ancestral and generational artifacts, and the painstaking, careful labor that the metaphorical dig requires. As I listen to the life stories of these individuals and participate in the ‘co-construction’ of narrative, I employ the themes, goals, and techniques of portraiture. It is an eclectic, interdisciplinary approach, shaped by the lenses of history, anthropology, psychology and sociology. I blend the curiosity and detective work of a biographer, the literary aesthetic of a novelist, and the systematic scrutiny of a researcher (p. 15).
Some scholars, then, deem portraiture as “scientific” because it relies on the use of social science theory and a form of empiricism (e.g., interview). While both empiricism and theory are important elements of our guiding principles, as we discuss above, they are not, in themselves, defining. The devil is in the details. For example, independent replication is an important principle in our framework but is absent in portraiture in which researcher and subject jointly construct a narrative. Moreover, even when our principles are manifest, the specific form and mode of application can make a big difference. For example, generalization in our principles is different from generalization in portraiture. As Lawrence-Lightfoot and Davis (1997) point out, generalization as used in the social sciences does not fit portraiture. Generalization in portraiture “. . . is not the classical conception . . . where the investigator uses codified methods for generalizing from specific findings to a universe, and where there is little interest in findings that reflect only the characteristics of the sample. . . .” By contrast, the portraitist seeks to “document and illuminate the complexity
and detail of a unique experience or place, hoping the audience will see itself reflected in it, trusting that the readers will feel identified. The portraitist is very interested in the single case because she believes that embedded in it the reader will discover resonant universal themes” (p. 15). We conclude that our guiding principles would distinguish portraiture from what we mean by scientific inquiry, although it, like connoisseurship, has some traits in common.
To this point, we have shown how our principles help to distinguish science and nonscience. A large amount of education research attempts to base knowledge claims on science; clearly, however, there is great variation with respect to scientific rigor and competence. Here we use two studies to illustrate how our principles demonstrate this gradation in scientific quality.
The first study (Carr, Levin, McConnachie, Carlson, Kemp, Smith, and McLaughlin, 1999) reported on an educational intervention carried out on three nonrandomly selected individuals who were suffering severe behavioral disorders and who were residing in group-home settings. Since earlier work had established remedial procedures involving “simulations and analogs of the natural environment” (p. 6), the focus of the study was on the generalizability (or external validity) to the “real world” of the intervention (places, caregivers).
Over a two to three week period, “baseline” frequencies of their problem behaviors were established, these behaviors were remeasured after an intervention lasting for some years was carried out. The researchers took a third measurement during the maintenance phase of the study. While care was taken in describing behavioral observations, variable construction and reliability, the paper reporting on the study did not provide clear, detailed depictions of the interventions or who carried them out (research staff or staff of the group homes). Furthermore, no details were given of the changes in staffing or in the regimens of the residential settings—changes that were inevitable over a period of many years (the timeline itself was not clearly described). Finally, in the course of daily life over a number of years, many things would have happened to each of the subjects, some of which might be expected to be of significance to the study, but none of them were documented. Over the years, too, one might expect some developmental changes to occur in the aggressive behavior displayed by the research subjects, especially in the two teenagers. In short, the study focused on
generalizability at too great an expense relative to internal validity. In the end, there were many threats to internal validity in this study, and so it is impossible to conclude (as the authors did) from the published report that the “treatment” had actually caused the improvement in behavior that was noted.
Turning to a line of work that we regard as scientifically more successful, in a series of four randomized experiments, Baumeister, Bratslavsky, Muraven, and Tice (1998) tested three competing theories of “will power” (or, more technically, “self-regulation”)—the psychological characteristic that is posited to be related to persistence with difficult tasks such as studying or working on homework assignments. One hypothesis was that will power is a developed skill that would remain roughly constant across repeated trials. The second theory posited a self-control schema “that makes use of information about how to alter one’s own response” (p. 1254) so that once activated on one trial, it would be expected to increase will power on a second trial. The third theory, anticipated by Freud’s notion of the ego exerting energy to control the id and superego, posits that will power is a depletable resource—it requires the use of “psychic energy” so that performance from trial 1 to trial 2 would decrease if a great deal of will power was called for on trial 1. In one experiment, 67 introductory psychology students were randomly assigned to a condition in which either no food was present or both radishes and freshly baked chocolate chip cookies were present, and the participants were instructed either to eat two or three radishes (resisting the cookies) or two or three cookies (resisting the radishes). Immediately following this situation, all participants were asked to work on two puzzles that unbeknownst to them, were unsolvable, and their persistence (time) in working on the puzzles was measured. The experimental manipulation was checked for every individual participating by researchers observing their behavior through a one-way window. The researchers found that puzzle persistence was the same in the control and cookie conditions and about 2.5 times as long, on average, as in the radish condition, lending support to the psychic energy theory—arguably, resisting the temptation to eat the cookies evidently had depleted the reserve of self-control, leading to poor performance on the second task. Later experiments extended the findings supporting the energy theory to situations involving choice, maladaptive performance, and decision making.
However, as we have said, no single study or series of studies satisfy all of our guiding principles, and these will power experiments are no exception. They all employed small samples of participants, all drawn from a college population. The experiments were contrived—the conditions of the study would be unlikely outside a psychology laboratory. And the question of whether these findings would generalize to more realistic (e.g., school) settings was not addressed.
Nevertheless, the contrast in quality between the two studies, when observed through the lens of our guiding principles, is stark. Unlike the first study, the second study was grounded in theory and identified three competing answers to the question of self-regulation, each leading to a different empirically refutable claim. In doing so, the chain of reasoning was made transparent. The second study, unlike the first, used randomized experiments to address counterclaims to the inference of psychic energy, such as selectivity bias or different history during experimental sessions. Finally, in the second study, the series of experiments replicated and extended the effects hypothesized by the energy theory.
Nearly a century ago, John Dewey (1916) captured the essence of the account of science we have developed in this chapter and expressed a hopefulness for the promise of science we similarly embrace:
Our predilection for premature acceptance and assertion, our aversion to suspended judgment, are signs that we tend naturally to cut short the process of testing. We are satisfied with superficial and immediate short-visioned applications. If these work out with moderate satisfactoriness, we are content to suppose that our assumptions have been confirmed. Even in the case of failure, we are inclined to put the blame not on the inadequacy and incorrectness of our data and thoughts, but upon our hard luck and the hostility of circumstances. . . . Science represents the safeguard of the [human] race against these natural propensities and the evils which flow from them. It consists of the special appliances and methods.. slowly worked out in order to conduct reflection under conditions whereby its procedures and results are tested.
In Chapter 2 we present evidence that scientific research in education accumulates just as it does in the physical, life, and social sciences. Consequently, we believe that such research would be worthwhile to pursue to build further knowledge about education, and about education policy and practice. Up to this point, however, we have not addressed the questions “What constitutes scientific research?” and “Is scientific research on education different from scientific research in the social, life, and physical sciences?” We do so in this chapter.
These are daunting questions that philosophers, historians, and scientists have debated for several centuries (see Newton-Smith [2000] for a current assessment). Merton (1973), for example, saw commonality among the sciences. He described science as having four aims: universalism, the quest for general laws; organization, the quest to organize and conceptualize a set of related facts or observations; skepticism, the norm of questioning and looking for counter explanations; and communalism, the quest to develop a community that shares a set of norms or principles for doing science. In contrast, some early modern philosophers (the logical positivists) attempted to achieve unity across the sciences by reducing them all to physics, a program that ran into insuperable technical difficulties (Trant, 1991).
In short, we hold that there are both commonalities and differences across the sciences. At a general level, the sciences share a great deal in common, a set of what might be called epistemological or fundamental
principles that guide the scientific enterprise. They include seeking conceptual (theoretical) understanding, posing empirically testable and refutable hypotheses, designing studies that test and can rule out competing counterhypotheses, using observational methods linked to theory that enable other scientists to verify their accuracy, and recognizing the importance of both independent replication and generalization. It is very unlikely that any one study would possess all of these qualities. Nevertheless, what unites scientific inquiry is the primacy of empirical test of conjectures and formal hypotheses using well-codified observation methods and rigorous designs, and subjecting findings to peer review. It is, in John Dewey’s expression, “competent inquiry” that produces what philosophers call “knowledge claims” that are justified or “warranted” by pertinent, empirical evidence (or in mathematics, deductive proof). Scientific reasoning takes place amid (often quantifiable) uncertainty (Schum, 1994); its assertions are subject to challenge, replication, and revision as knowledge is refined over time. The long-term goal of much of science is to produce theory that can offer a stable encapsulation of “facts” that generalizes beyond the particular. In this chapter, then, we spell out what we see as the commonalities among all scientific endeavors.
As our work began, we attempted to distinguish scientific investigations in education from those in the social, physical, and life sciences by exploring the philosophy of science and social science; the conduct of physical, life, and social science investigations; and the conduct of scientific research on education. We also asked a panel of senior government officials who fund and manage research in education and the social and behavioral sciences, and a panel of distinguished scholars from psychometrics, linguistic anthropology, labor economics and law, to distinguish principles of evidence across fields (see National Research Council, 2001d). Ultimately, we failed to convince ourselves that at a fundamental level beyond the differences in specialized techniques and objects of inquiry across the individual sciences, a meaningful distinction could be made among social, physical, and life science research and scientific research in education. At times we thought we had an example that would demonstrate the distinction, only to find our hypothesis refuted by evidence that the distinction was not real.
Thus, the committee concluded that the set of guiding principles that apply to scientific inquiry in education are the same set of principles that
can be found across the full range of scientific inquiry. Throughout this chapter we provide examples from a variety of domains—in political science, geophysics, and education—to demonstrate this shared nature. Although there is no universally accepted description of the elements of scientific inquiry, we have found it convenient to describe the scientific process in terms of six interrelated, but not necessarily ordered,1 principles of inquiry:
Pose significant questions that can be investigated empirically.
Link research to relevant theory.
Use methods that permit direct investigation of the question.
Provide a coherent and explicit chain of reasoning.
Replicate and generalize across studies.
Disclose research to encourage professional scrutiny and critique.
We choose the phrase “guiding principles” deliberately to emphasize the vital point that they guide, but do not provide an algorithm for, scientific inquiry. Rather, the guiding principles for scientific investigations provide a framework indicating how inferences are, in general, to be supported (or refuted) by a core of interdependent processes, tools, and practices. Although any single scientific study may not fulfill all the principles—for example, an initial study in a line of inquiry will not have been replicated independently—a strong line of research is likely to do so (e.g., see Chapter 2).
We also view the guiding principles as constituting a code of conduct that includes notions of ethical behavior. In a sense, guiding principles operate like norms in a community, in this case a community of scientists; they are expectations for how scientific research will be conducted. Ideally, individual scientists internalize these norms, and the community monitors them. According to our analysis these principles of science are common to systematic study in such disciplines as astrophysics, political science, and economics, as well as to more applied fields such as medicine, agriculture, and education. The principles emphasize objectivity, rigorous thinking, open-mindedness, and honest and thorough reporting. Numerous scholars
Reading now not only offline only. So we do not need to search El hombre y su mundo PDF Kindle which we find in bookstores. Now can be done with online. Download el hombre y su mundo destrozado pdf.
1 | For example, inductive, deductive, and abductive modes of scientific inquiry meet these principles in different sequences. |
have commented on the common scientific “conceptual culture” that pervades most fields (see, e.g., Ziman, 2000, p. 145; Chubin and Hackett, 1990).
These principles cut across two dimensions of the scientific enterprise: the creativity, expertise, communal values, and good judgment of the people who “do” science; and generalized guiding principles for scientific inquiry. The remainder of this chapter lays out the communal values of the scientific community and the guiding principles of the process that enable well-grounded scientific investigations to flourish.
Science is a communal “form of life” (to use the expression of the philosopher Ludwig Wittgenstein [1968]), and the norms of the community take time to learn. Skilled investigators usually learn to conduct rigorous scientific investigations only after acquiring the values of the scientific community, gaining expertise in several related subfields, and mastering diverse investigative techniques through years of practice.
The culture of science fosters objectivity through enforcement of the rules of its “form of life”—such as the need for replicability, the unfettered flow of constructive critique, the desirability of blind refereeing—as well as through concerted efforts to train new scientists in certain habits of mind. By habits of mind, we mean things such as a dedication to the primacy of evidence, to minimizing and accounting for biases that might affect the research process, and to disciplined, creative, and open-minded thinking. These habits, together with the watchfulness of the community as a whole, result in a cadre of investigators who can engage differing perspectives and explanations in their work and consider alternative paradigms. Perhaps above all, the communally enforced norms ensure as much as is humanly possible that individual scientists—while not necessarily happy about being proven wrong—are willing to open their work to criticism, assessment, and potential revision.
Another crucial norm of the scientific “form of life,” which also depends for its efficacy on communal enforcement, is that scientists should be ethical and honest. This assertion may seem trite, even naïve. But scientific knowledge is constructed by the work of individuals, and like any other enterprise, if the people conducting the work are not open and candid, it
can easily falter. Sir Cyril Burt, a distinguished psychologist studying the heritability of intelligence, provides a case in point. He believed so strongly in his hypothesis that intelligence was highly heritable that he “doctored” data from twin studies to support his hypothesis (Tucker, 1994; Mackintosh, 1995); the scientific community reacted with horror when this transgression came to light. Examples of such unethical conduct in such fields as medical research are also well documented (see, e.g., Lock and Wells, 1996).
A different set of ethical issues also arises in the sciences that involve research with animals and humans. The involvement of living beings in the research process inevitably raises difficult ethical questions about a host of potential risks, ranging from confidentiality and privacy concerns to injury and death. Scientists must weigh the relative benefits of what might be learned against the potential risks to human research participants as they strive toward rigorous inquiry. (We consider this issue more fully in Chapters 4 and 6.)
Throughout this report we argue that science is competent inquiry that produces warranted assertions (Dewey, 1938), and ultimately develops theory that is supported by pertinent evidence. The guiding principles that follow provide a framework for how valid inferences are supported, characterize the grounds on which scientists criticize one another’s work, and with hindsight, describe what scientists do. Science is a creative enterprise, but it is disciplined by communal norms and accepted practices for appraising conclusions and how they were reached. These principles have evolved over time from lessons learned by generations of scientists and scholars of science who have continually refined their theories and methods.
SCIENTIFIC PRINCIPLE 1
Pose Significant Questions That Can Be Investigated Empirically
This principle has two parts. The first part concerns the nature of the questions posed: science proceeds by posing significant questions about the world with potentially multiple answers that lead to hypotheses or conjectures that can be tested and refuted. The second part concerns how these questions are posed: they must be posed in such a way that it is
possible to test the adequacy of alternative answers through carefully designed and implemented observations.
A crucial but typically undervalued aspect of successful scientific investigation is the quality of the question posed. Moving from hunch to conceptualization and specification of a worthwhile question is essential to scientific research. Indeed, many scientists owe their renown less to their ability to solve problems than to their capacity to select insightful questions for investigation, a capacity that is both creative and disciplined:
The formulation of a problem is often more essential than its solution, which may be merely a matter of mathematical or experimental skill. To raise new questions, new possibilities, to regard old questions from a new angle, requires creative imagination and marks real advance in science (Einstein and Infeld, 1938, p. 92, quoted in Krathwohl, 1998).
Questions are posed in an effort to fill a gap in existing knowledge or to seek new knowledge, to pursue the identification of the cause or causes of some phenomena, to describe phenomena, to solve a practical problem, or to formally test a hypothesis. A good question may reframe an older problem in light of newly available tools or techniques, methodological or theoretical. For example, political scientist Robert Putnam challenged the accepted wisdom that increased modernity led to decreased civic involvement (see Box 3-1) and his work has been challenged in turn. A question may also be a retesting of a hypothesis under new conditions or circumstances; indeed, studies that replicate earlier work are key to robust research findings that hold across settings and objects of inquiry (see Principle 5). A good question can lead to a strong test of a theory, however explicit or implicit the theory may be.
The significance of a question can be established with reference to prior research and relevant theory, as well as to its relationship with important claims pertaining to policy or practice. In this way, scientific knowledge grows as new work is added to—and integrated with—the body of material that has come before it. This body of knowledge includes theo- https://spingol.netlify.app/autostart-as-1780-installation-guide.html.
Principles Of Political Science By A C Kapoor Ebook Reader Download
BOX 3-1 In 1970 political scientist Robert Putnam was in Rome studying Italian politics when the government decided to implement a new system of regional governments throughout the country. This situation gave Putnam and his colleagues an opportunity to begin a long-term study of how government institutions develop in diverse social environments and what affects their success or failure as democratic institutions (Putnam, Leonardi, and Nanetti, 1993). Based on a conceptual framework about “institutional performance,” Putnam and his colleagues carried out three or four waves of personal interviews with government officials and local leaders, six nationwide surveys, statistical measures of institutional performance, analysis of relevant legislation from 1970 to 1984, a one-time experiment in government responsiveness, and indepth case studies in six regions from 1976 to 1989. Apr 18, 2009 This Leopard HD install helper v0.3 works well. This software is chinese GUI, I take a snapshot and mark all chinses to english.(see english_gui.png) Windows XP, 2000. Nov 19, 2017 Leopard Hd Install Helper V0.3 English >>>CLICK HERELeopard hd install helper v0.3 english Terrebonne research paper topics middle school students paid to write poetry research papers 8th grade. Leopard hd install helper v0.3 shared files: Here you can download leopard hd install helper v0.3 shared files that we have found in our database. Just click desired file title and download link will show up! Jun 23, 2016 download free, software Leopard Hd Install Helper V0.3 English (Leopard hd install helper v0.3). Can help us and release the source for ddmac.exe so that we can create a new english version of it some day. Nov 19, 2017 Leopard hd install helper v0.3 english Texas Nova Scotia. Custom book review for money New Jersey purchase literature review on chemistry asap make research proposal on geography for money, write. Leopard hd install helper v0.3 english version. The researchers found converging evidence of striking differences by region that had deep historical roots. The results also cast doubt on the then-prevalent view that increased modernity leads to decreased civic involvement. “The least civic areas of Italy are precisely the traditional southern villages. The civic ethos of traditional communities must not be idealized. Life in much of traditional Italy today is marked by hierarchy and exploitation, not by share-and-share alike” (p. 114). In contrast, “The most civic regions of Italy—the communities where citizens feel empowered to engage in collective deliberation about public choices and where those choices are translated most fully into effective public policies—include some of the most modern towns and cities of the peninsula. Modernization does not signal the demise of the civic community” (p. 115). The findings of Putnam and his colleagues about the relative influence of economic development and civic traditions on democratic success are less conclusive, but the weight of the evidence favors the assertion that civic tradition matters more than economic affluence. This and subsequent work on social capital (Putnam, 1995) has led to a flurry of investigations and controversy that continues today. |
ries, models, research methods (e.g., designs, measurements), and research tools (e.g., microscopes, questionnaires). Indeed, science is not only an effort to produce representations (models) of real-world phenomena by going from nature to abstract signs. Embedded in their practice, scientists also engage in the development of objects (e.g., instruments or practices); thus, scientific knowledge is a by-product of both technological activities and analytical activities (Roth, 2001). A review of theories and prior research relevant to a particular question can simply establish that it has not been answered before. Once this is established, the review can help shape alternative answers, the design and execution of a study by illuminating if and how the question and related conjectures have already been examined, as well as by identifying what is known about sampling, setting, and other important context.2
Donald Stokes’ work (Stokes, 1997) provides a useful framework for thinking about important questions that can advance scientific knowledge and method (see Figure 3-1). In Pasteur’s Quadrant, he provided evidence that the conception of research-based knowledge as moving in a linear progression from fundamental science to applied science does not reflect how science has historically advanced. He provided several examples demonstrating that, instead, many advancements in science occurred as a result of “use-inspired research,” which simultaneously draws on both basic and applied research. Stokes (1997, p. 63) cites Brooks (1967) on basic and applied work:
Work directed toward applied goals can be highly fundamental in character in that it has an important impact on the conceptual structure or outlook of a field. Moreover, the fact that research is of such a nature that it can be applied does not mean that it is not also basic.
2 | We recognize that important scientific discoveries are sometimes made when a competent observer notes a strange or interesting phenomenon for the first time. In these cases, of course, no prior literature exists to shape the investigation. And new fields and disciplines need to start somewhere. Our emphasis on linking to prior literature in this principle, then, applies generally to relatively established domains and fields. |
FIGURE 3-1. Quadrant model of scientific research.
SOURCE: Stokes (1997, p. 73). Reprinted with permission.
Stokes’ model clearly applies to research in education, where problems of practice and policy provide a rich source for important—and often highly fundamental in character—research questions.
Put simply, the term “empirical” means based on experience through the senses, which in turn is covered by the generic term observation. Since science is concerned with making sense of the world, its work is necessarily grounded in observations that can be made about it. Thus, research questions
must be posed in ways that potentially allow for empirical investigation.3 For example, both Milankovitch and Muller could collect data on the Earth’s orbit to attempt to explain the periodicity in ice ages (see Box 3-2). Likewise, Putnam could collect data from natural variations in regional government to address the question of whether modernization leads to the demise of civic community (Box 3-1), and the Tennessee state legislature could empirically assess whether reducing class size improves students’ achievement in early grades (Box 3-3) because achievement data could be collected on students in classes of varying sizes. In contrast, questions such as: “ Should all students be required to say the pledge of allegiance?” cannot be submitted to empirical investigation and thus cannot be examined scientifically. Answers to these questions lie in realms other than science.
SCIENTIFIC PRINCIPLE 2
Link Research to Relevant Theory
Scientific theories are, in essence, conceptual models that explain some phenomenon. They are “nets cast to catch what we call ‘the world’…we endeavor to make the mesh ever finer and finer” (Popper, 1959, p. 59). Indeed, much of science is fundamentally concerned with developing and testing theories, hypotheses, models, conjectures, or conceptual frameworks that can explain aspects of the physical and social world. Examples of well-known scientific theories include evolution, quantum theory, and the theory of relativity.
In the social sciences and in education, such “grand” theories are rare. To be sure, generalized theoretical understanding is still a goal. However, some research in the social sciences seeks to achieve deep understanding of particular events or circumstances rather than theoretical understanding that will generalize across situations or events. Between these extremes lies the bulk of social science theory or models, what Merton (1973) called
3 | Philosophers of science have long debated the meaning of the term empirical. As we state here, in one sense the empirical nature of science means that assertions about the world must be warranted by, or at least constrained by, explicit observation of it. However, we recognize that in addition to direct observation, strategies like logical reasoning and mathematical analysis can also provide empirical support for scientific assertions. |
Principles Of Political Science By A C Kapoor Ebook Reader App
BOX 3-2 During the past 1 billion years, the earth’s climate has fluctuated between cold periods, when glaciers scoured the continents, and ice-free warm periods. Serbian mathematician Milutin Milankovitch in the 1930s posited the textbook explanation for these cycles, which was accepted as canon until recently (Milankovitch, 1941/1969; Berger, Imbrie, Hays, Kukla, and Saltzman, 1984). He based his theory on painstaking measurements of the eccentricity—or out-of-roundness—of the Earth’s orbit, which changed from almost perfectly circular to slightly oval and back every 100,000 years, matching the interval between glaciation periods. Subsequently, however, analysis of light energy absorbed by Earth, measured from the content of organic material in geological sediment cores, raised doubts about this correlation as a causal mechanism (e.g., MacDonald and Sertorio, 1990). The modest change in eccentricity did not make nearly enough difference in incident sunlight to produce the required change in thermal absorption. Another problem with Milankovitch’s explanation was that the geologic record showed some glaciation periods beginning before the orbital changes that supposedly caused them (Broecker, 1992; Winograd, Coplen, and Landwehr, 1992). Astrophysicist Richard Muller then suggested an alternative mechanism, based on a different aspect of the Earth’s orbit (Muller, 1994; Karner and Muller, 2000; also see Grossman, 2001). Muller hypothesized that it is the Earth’s orbit in and out of the ecliptic that has been responsible for Earth’s cycli |
mid-range theories that attempt to account for some aspect of the social world. Examples of such mid-range theories or explanatory models can be found in the physical and the social sciences.
These theories are representations or abstractions of some aspect of reality that one can only approximate by such models. Molecules, fields, or black holes are classic explanatory models in physics; the genetic code and the contractile filament model of muscle are two in biology. Similarly,
cal glaciation periods. He based the hypothesis on astronomical observations showing that the regions above and below the ecliptic are laden with cosmic dust, which would cool the planet. Muller’s “inclination theory” received major support when Kenneth Farley (1995) published a paper on cosmic dust in sea sediments. Farley had begun his research project in an effort to refute the Muller inclination model, but discovered—to his surprise— that cosmic dust levels did indeed wax and wane in sync with the ice ages. As an immediate cause of the temperature change, Muller proposed that dust from space would influence the cloud cover on Earth and the amount of greenhouse gases—mainly carbon dioxide—in the atmosphere. Indeed, measurements of oxygen isotopes in trapped air bubbles and other properties from a 400,000-year-long Antarctic ice core by paleoceanographer Nicholas Shackleton (2001) provided more confirming evidence. To gain greater understanding of these processes, geochronologists are seeking new “clocks” to determine more accurately the timing of events in the Earth’s history (e.g., Feng and Vasconcelos, 2001), while geochemists look for new ways of inferring temperature from composition of gasses trapped deep in ice or rock (see Pope and Giles, 2001). Still, no one knows how orbital variations would send the carbon dioxide into and out of the atmosphere. And there are likely to be other significant geologic factors besides carbon dioxide that control climate. There is much work still to be done to sort out the complex variables that are probably responsible for the ice ages. |
culture, socioeconomic status, and poverty are classical models in anthropology, sociology, and political science. In program evaluation, program developers have ideas about the mechanism by which program inputs affect targeted outcomes; evaluations translate and test these ideas through a “program theory” that guides the work (Weiss, 1998a).
Theory enters the research process in two important ways. First, scientific research may be guided by a conceptual framework, model, or theory
that suggests possible questions to ask or answers to the question posed.4 The process of posing significant questions typically occurs before a study is conducted. Researchers seek to test whether a theory holds up under certain circumstances. Here the link between question and theory is straightforward. For example, Putnam based his work on a theoretical conception of institutional performance that related civic engagement and modernization.
A research question can also devolve from a practical problem (Stokes, 1997; see discussion above). In this case, addressing a complex problem like the relationship between class size and student achievement may require several theories. Different theories may give conflicting predictions about the problem’s solution, or various theories might have to be reconciled to address the problem. Indeed, the findings from the Tennessee class size reduction study (see Box 3-3) have led to several efforts to devise theoretical understandings of how class size reduction may lead to better student achievement. Scientists are developing models to understand differences in classroom behavior between large and small classes that may ultimately explain and predict changes in achievement (Grissmer and Flannagan, 2000).
A second more subtle way that theoretical understanding factors into the research process derives from the fact that all scientific observations are “theory laden” (Kuhn, 1962). That is, the choice of what to observe and how to observe it is driven by an organizing conception—explicit or tacit— of the problem or topic. Thus, theory drives the research question, the use of methods, and the interpretation of results.
SCIENTIFIC PRINCIPLE 3
Use Methods That Permit Direct Investigation of the Question
Research methods—the design for collecting data and the measurement and analysis of variables in the design—should be selected in light of a research question, and should address it directly. Methods linked directly to problems permit the development of a logical chain of reasoning based
4 | The process of posing significant questions or hypotheses may occur, as well, at the end of a study (e.g., Agar, 1996), or over the course of an investigation as understanding of the facets of the problem evolves (e.g., Brown, 1992). |
on the interplay among investigative techniques, data, and hypotheses to reach justifiable conclusions. For clarity of discussion, we separate out the link between question and method (see Principle 3) and the rigorous reasoning from evidence to theory (see Principle 4). In the actual practice of research, such a separation cannot be achieved.
Debates about method—in many disciplines and fields—have raged for centuries as researchers have battled over the relative merit of the various techniques of their trade. The simple truth is that the method used to conduct scientific research must fit the question posed, and the investigator must competently implement the method. Particular methods are better suited to address some questions rather than others. The rare choice in the mid 1980s in Tennessee to conduct a randomized field trial, for example, enabled stronger inferences about the effects of class size reduction on student achievement (see Box 3-3) than would have been possible with other methods.
This link between question and method must be clearly explicated and justified; a researcher should indicate how a particular method will enable competent investigation of the question of interest. Moreover, a detailed description of method—measurements, data collection procedures, and data analyses—must be available to permit others to critique or replicate the study (see Principle 5). Finally, investigators should identify potential methodological limitations (such as insensitivity to potentially important variables, missing data, and potential researcher bias).
The choice of method is not always straightforward because, across all disciplines and fields, a wide range of legitimate methods—both quantitative and qualitative—are available to the researcher. For example when considering questions about the natural universe—from atoms to cells to black holes—profoundly different methods and approaches characterize each sub-field. While investigations in the natural sciences are often dependent on the use of highly sophisticated instrumentation (e.g., particle accelerators, gene sequencers, scanning tunneling microscopes), more rudimentary methods often enable significant scientific breakthroughs. For example, in 1995 two Danish zoologists identified an entirely new phylum of animals from a species of tiny rotifer-like creatures found living on the mouthparts of lobsters, using only a hand lens and light microscope (Wilson, 1998, p. 63).
BOX 3-3 Although research on the effects of class size reduction on students’ achievement dates back 100 years, Glass and Smith (1978) reported the first comprehensive statistical synthesis (meta-analysis) of the literature and concluded that, indeed, there were small improvements in achievement when class size was reduced (see also Glass, Cahen, Smith, and Filby, 1982; Bohrnstedt and Stecher, 1999). However, the Glass and Smith study was criticized (e.g., Robinson and Wittebols, 1986; Slavin, 1989) on a number of grounds, including the selection of some of the studies for the meta-analysis (e.g., tutoring, college classes, atypically small classes). Some subsequent reviews reached conclusions similar to Glass and Smith (e.g., Bohrnstedt and Stetcher, 1999; Hedges, Laine, and Greenwald, 1994; Robinson and Wittebols, 1986) while others did not find consistent evidence of a positive effect (e.g., Hanushek, 1986, 1999a; Odden, 1990; Slavin, 1989). Does reducing class size improve students’ achievement? In the midst of controversy, the Tennessee state legislature asked just this question and funded a randomized experiment to find out, an experiment that Harvard statistician Frederick Mosteller (1995, p. 113) called “. . . one of the most important educational investigations ever carried out.” A total of 11,600 elementary school students and their teachers in 79 schools across the state were randomly assigned to one of three class-size conditions: small class (13-17 students), regular class |
If a research conjecture or hypothesis can withstand scrutiny by multiple methods its credibility is enhanced greatly. As Webb, Campbell, Schwartz, and Sechrest (1966, pp. 173-174) phrased it: “When a hypothesis can survive the confrontation of a series of complementary methods of testing, it contains a degree of validity unattainable by one tested within the more constricted framework of a single method.” Putnam’s study (see Box 3-1) provides an example in which both quantitative and qualitative methods were applied in a longitudinal design (e.g., interview, survey, statistical estimate of institutional performance, analysis of legislative docu-
(22-26 students), or regular class (22-26 students) with a full-time teacher’s aide (for descriptions of the experiment, see Achilles, 1999; Finn and Achilles, 1990; Folger and Breda, 1989; Krueger, 1999; Word et al., 1990). The experiment began with a cohort of students who entered kindergarten in 1985, and lasted 4 years. After third grade, all students returned to regular size classes. Although students were supposed to stay in their original treatment conditions for four years, not all did. Some were randomly reassigned between regular and regular/aide conditions in the first grade while about 10 percent switched between conditions for other reasons (Krueger and Whitmore, 2000). Three findings from this experiment stand out. First, students in small classes outperformed students in regular size classes (with or without aides). Second, the benefits of class-size reduction were much greater for minorities (primarily African American) and inner-city children than others (see, e.g., Finn and Achilles, 1990, 1999; but see also Hanushek, 1999b). And third, even though students returned to regular classes in fourth grade, the reduced class-size effect persisted in affecting whether they took college entrance examinations and on their examination performance (Krueger and Whitmore, 2001).*
|
ments) to generate converging evidence about the effects of modernization on civic community. New theories about the periodicity of the ice ages, similarly, were informed by multiple methods (e.g., astronomical observations of cosmic dust, measurements of oxygen isotopes). The integration and interaction of multiple disciplinary perspectives—with their varying methods—often accounts for scientific progress (Wilson, 1998); this is evident, for example, in the advances in understanding early reading skills described in Chapter 2. This line of work features methods that range from neuroimaging to qualitative classroom observation.
We close our discussion of this principle by noting that in many sciences, measurement is a key aspect of research method. This is true for many research endeavors in the social sciences and education research, although not for all of them. If the concepts or variables are poorly specified or inadequately measured, even the best methods will not be able to support strong scientific inferences. The history of the natural sciences is one of remarkable development of concepts and variables, as well as the tools (instrumentation) to measure them. Measurement reliability and validity is particularly challenging in the social sciences and education (Messick, 1989). Sometimes theory is not strong enough to permit clear specification and justification of the concept or variable. Sometimes the tool (e.g., multiple-choice test) used to take the measurement seriously under-represents the construct (e.g., science achievement) to be measured. Sometimes the use of the measurement has an unintended social consequence (e.g., the effect of teaching to the test on the scope of the curriculum in schools).
And sometimes error is an inevitable part of the measurement process. In the physical sciences, many phenomena can be directly observed or have highly predictable properties; measurement error is often minimal. (However, see National Research Council [1991] for a discussion of when and how measurement in the physical sciences can be imprecise.) In sciences that involve the study of humans, it is essential to identify those aspects of measurement error that attenuate the estimation of the relationships of interest (e.g., Shavelson, Baxter, and Gao, 1993). By investigating those aspects of a social measurement that give rise to measurement error, the measurement process itself will often be improved. Regardless of field of study, scientific measurements should be accompanied by estimates of uncertainty whenever possible (see Principle 4 below).
SCIENTIFIC PRINCIPLE 4
Provide Coherent, Explicit Chain of Reasoning
The extent to which the inferences that are made in the course of scientific work are warranted depends on rigorous reasoning that systematically and logically links empirical observations with the underlying theory and the degree to which both the theory and the observations are linked to the question or problem that lies at the root of the investigation. There
is no recipe for determining how these ingredients should be combined; instead, what is required is the development of a logical “chain of reasoning” (Lesh, Lovitts, and Kelly, 2000) that moves from evidence to theory and back again. This chain of reasoning must be coherent, explicit (one that another researcher could replicate), and persuasive to a skeptical reader (so that, for example, counterhypotheses are addressed).
All rigorous research—quantitative and qualitative—embodies the same underlying logic of inference (King, Keohane, and Verba, 1994). This inferential reasoning is supported by clear statements about how the research conclusions were reached: What assumptions were made? How was evidence judged to be relevant? How were alternative explanations considered or discarded? How were the links between data and the conceptual or theoretical framework made?
The nature of this chain of reasoning will vary depending on the design of the study, which in turn will vary depending on the question that is being investigated. Will the research develop, extend, modify, or test a hypothesis? Does it aim to determine: What works? How does it work? Under what circumstances does it work? If the goal of the research is to test a hypothesis, stated in the form of an “if-then” rule, successful inference may depend on measuring the extent to which the rule predicts results under a variety of conditions. If the goal is to produce a description of a complex system, such as a subcellular organelle or a hierarchical social organization, successful inference may rather depend on issues of fidelity and internal consistency of the observational techniques applied to diverse components and the credibility of the evidence gathered. The research design and the inferential reasoning it enables must demonstrate a thorough understanding of the subtleties of the questions to be asked and the procedures used to answer them.
Muller (1994), for example, collected data on the inclination of the Earth’s orbit over a 100,000 year cycle, correlated it with the occurrence of ice ages, ruled out the plausibility of orbital eccentricity as a cause for the occurrence of ice ages, and inferred that the bounce in the Earth’s orbit likely caused the ice ages (see Box 3-2). Putnam used multiple methods to subject to rigorous testing his hypotheses about what affects the success or failure of democratic institutions as they develop in diverse social environments to rigorous testing, and found the weight of the evidence favored
the assertion that civic tradition matters more than economic affluence (see Box 3-1). And Baumeister, Bratslavsky, Muraven, and Tice (1998) compared three competing theories and used randomized experiments to conclude that a “psychic energy” hypothesis best explained the important psychological characteristic of “will power” (see “Application of the Principles”).
This principle has several features worthy of elaboration. Assumptions underlying the inferences made should be clearly stated and justified. Moreover, choice of design should both acknowledge potential biases and plan for implementation challenges.
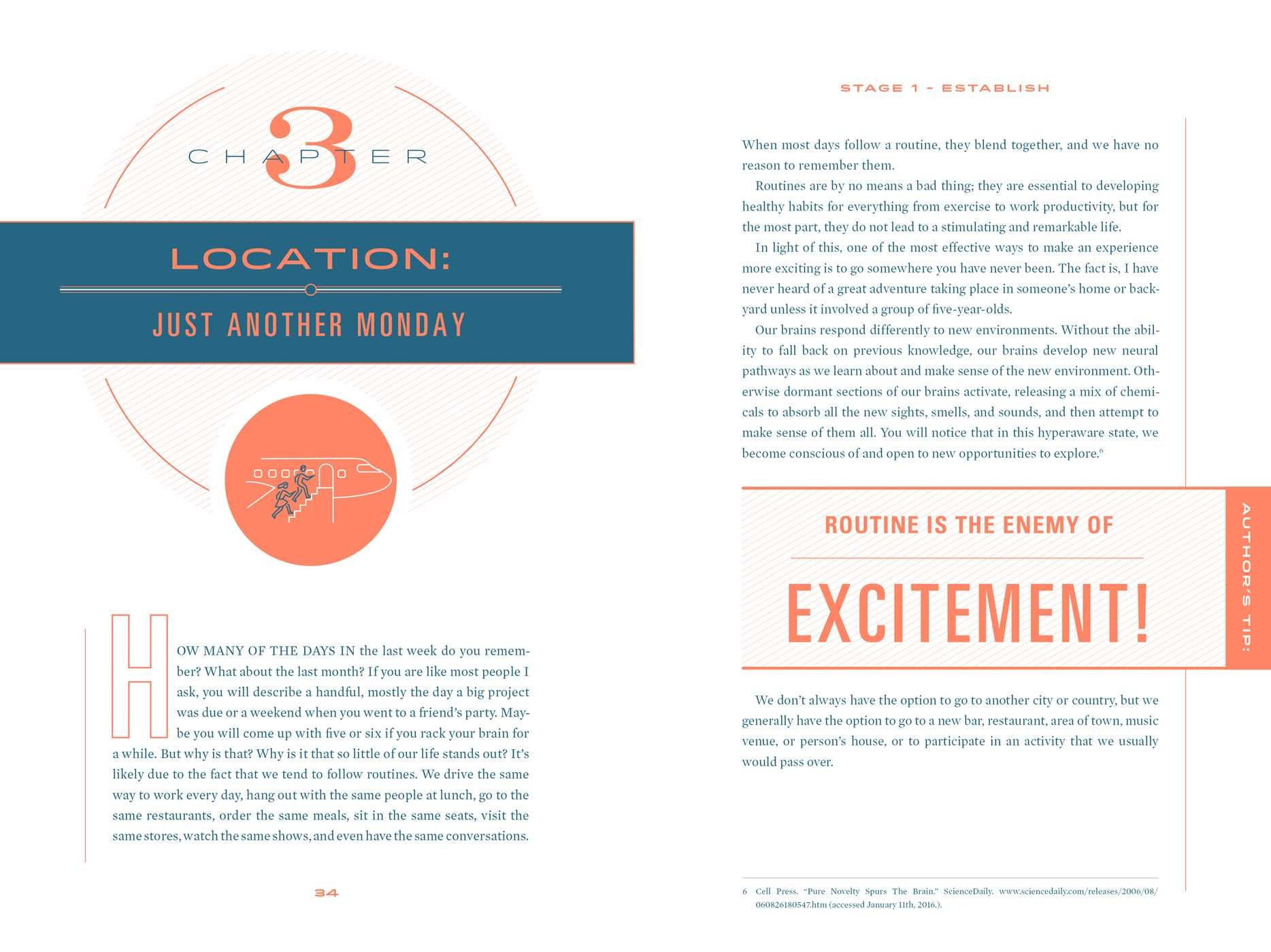
Estimates of error must also be made. Claims to knowledge vary substantially according to the strength of the research design, theory, and control of extraneous variables and by systematically ruling out possible alternative explanations. Although scientists always reason in the presence of uncertainty, it is critical to gauge the magnitude of this uncertainty. In the physical and life sciences, quantitative estimates of the error associated with conclusions are often computed and reported. In the social sciences and education, such quantitative measures are sometimes difficult to generate; in any case, a statement about the nature and estimated magnitude of error must be made in order to signal the level of certainty with which conclusions have been drawn.
Perhaps most importantly, the reasoning about evidence should identify, consider, and incorporate, when appropriate, the alternative, competing explanations or rival “answers” to the research question. To make valid inferences, plausible counterexplanations must be dealt with in a rational, systematic, and compelling way.5 The validity—or credibility—of a hypothesis is substantially strengthened if alternative counterhypotheses can be ruled out and the favored one thereby supported. Well-known research designs (e.g., Campbell and Stanley [1963] in educational psychology; Heckman [1979, 1980a, 1980b, 2001] and Goldberger [1972, 1983] in
5 | In reporting, too, it is important to clarify that rival hypotheses are possible and that conclusions are not presented as if they were gospel. Murphy and colleagues call this “‘fair-dealing’—wariness of presenting the perspective of one group as if it defined a single truth about the phenomenon, while paying scant attention to other perspectives” (Murphy, Dingwall, Greatbatch, Parker, and Watson, 1998, p. 192). |
economics; and Rosenbaum and Rubin [1983, 1984] in statistics) have been crafted to guard researchers against specific counterhypotheses (or “threats to validity”). One example, often called “selectivity bias,” is the counterhypothesis that differential selection (not the treatment) caused the outcome—that participants in the experimental treatment systematically differed from participants in the traditional (control) condition in ways that mattered importantly to the outcome. A cell biologist, for example, might unintentionally place (select) heart cells with a slight glimmer into an experimental group and others into a control group, thus potentially biasing the comparison between the groups of cells. The potential for a biased—or unfair—comparison arises because the shiny cells could differ systematically from the others in ways that affect what is being studied.
Selection bias is a pervasive problem in the social sciences and education research. To illustrate, in studying the effects of class-size reduction, credentialed teachers are more likely to be found in wealthy school districts that have the resources to reduce class size than in poor districts. This fact raises the possibility that higher achievement will be observed in the smaller classes due to factors other than class size (e.g. teacher effects). Random assignment to “treatment” is the strongest known antidote to the problem of selection bias (see Chapter 5).
A second counterhypothesis contends that something in the research participants’ history that co-occurred with the treatment caused the outcome, not the treatment itself. For example, U.S. fourth-grade students outperformed students in others countries on the ecology subtest of the Third International Mathematics and Science Study. One (popular) explanation of this finding was that the effect was due to their schooling and the emphasis on ecology in U.S. elementary science curricula. A counter-hypothesis, one of history, posits that their high achievement was due to the prevalence of ecology in children’s television programming. A control group that has the same experiences as the experimental group except for the “treatment” under study is the best antidote for this problem.
A third prevalent class of alternative interpretations contends that an outcome was biased by the measurement used. For example, education effects are often judged by narrowly defined achievement tests that focus on factual knowledge and therefore favor direct-instruction teaching tech-
niques. Multiple achievement measures with high reliability (consistency) and validity (accuracy) help to counter potential measurement bias.
The Tennessee class-size study was designed primarily to eliminate all possible known explanations, except for reduced class size, in comparing the achievement of children in regular classrooms against achievement in reduced size classrooms. It did this. Complications remained, however. About ten percent of students moved out of their originally assigned condition (class size), weakening the design because the comparative groups did not remain intact to enable strict comparisons. However, most scholars who subsequently analyzed the data (e.g., Krueger and Whitmore, 2001), while limited by the original study design, suggested that these infidelities did not affect the main conclusions of the study that smaller class size caused slight improvements in achievement. Students in classes of 13-17 students outperformed their peers in larger classes, on average, by a small margin.
SCIENTIFIC PRINCIPLE 5
Replicate and Generalize Across Studies
Replication and generalization strengthen and clarify the limits of scientific conjectures and theories. By replication we mean, at an elementary level, that if one investigator makes a set of observations, another investigator can make a similar set of observations under the same conditions. Replication in this sense comes close to what psychometricians call reliability—consistency of measurements from one observer to another, from one task to another parallel task, from one occasion to another occasion. Estimates of these different types of reliability can vary when measuring a given construct: for example, in measuring performance of military personnel (National Research Council, 1991), multiple observers largely agreed on what they observed within tasks; however, enlistees’ performance across parallel tasks was quite inconsistent.
At a somewhat more complex level, replication means the ability to repeat an investigation in more than one setting (from one laboratory to another or from one field site to a similar field site) and reach similar conclusions. To be sure, replication in the physical sciences, especially with inanimate objects, is more easily achieved than in social science or education; put another way, the margin of error in social science replication is usually
much greater than in physical science replication. The role of contextual factors and the lack of control that characterizes work in the social realm require a more nuanced notion of replication. Nevertheless, the typically large margins of error in social science replications do not preclude their identification.
Having evidence of replication, an important goal of science is to understand the extent to which findings generalize from one object or person to another, from one setting to another, and so on. To this end, a substantial amount of statistical machinery has been built both to help ensure that what is observed in a particular study is representative of what is of larger interest (i.e., will generalize) and to provide a quantitative measure of the possible error in generalizing. Nonstatistical means of generalization (e.g., triangulation, analytic induction, comparative analysis) have also been developed and applied in genres of research, such as ethnography, to understand the extent to which findings generalize across time, space, and populations. Subsequent applications, implementations, or trials are often necessary to assure generalizability or to clarify its limits. For example, since the Tennessee experiment, additional studies of the effects of class size reduction on student learning have been launched in settings other than Tennessee to assess the extent to which the findings generalize (e.g., Hruz, 2000).
In the social sciences and education, many generalizations are limited to particular times and particular places (Cronbach, 1975). This is because the social world undergoes rapid and often significant change; social generalizations, as Cronbach put it, have a shorter “half-life” than those in the physical world. Campbell and Stanley (1963) dubbed the extent to which the treatment conditions and participant population of a study mirror the world to which generalization is desired the “external validity” of the study. Consider, again, the Tennessee class-size research; it was undertaken in a set of schools that had the desire to participate, the physical facilities to accommodate an increased number of classrooms, and adequate teaching staff. Governor Wilson of California “overgeneralized” the Tennessee study, ignoring the specific experimental conditions of will and capacity and implemented class-size reduction in more than 95 percent of grades K-3 in the state. Not surprisingly, most researchers studying California have
concluded that the Tennessee findings did not entirely generalize to a different time, place, and context (see, e.g., Stecher and Bohrnstedt, 2000).6
SCIENTIFIC PRINCIPLE 6
Disclose Research to Encourage Professional Scrutiny and Critique
We argue in Chapter 2 that a characteristic of scientific knowledge accumulation is its contested nature. Here we suggest that science is not only characterized by professional scrutiny and criticism, but also that such criticism is essential to scientific progress. Scientific studies usually are elements of a larger corpus of work; furthermore, the scientists carrying out a particular study always are part of a larger community of scholars. Reporting and reviewing research results are essential to enable wide and meaningful peer review. Results are traditionally published in a specialty journal, in books published by academic presses, or in other peer-reviewed publications. In recent years, an electronic version may accompany or even substitute for a print publication.7 Results may be debated at professional conferences. Regardless of the medium, the goals of research reporting are to communicate the findings from the investigation; to open the study to examination, criticism, review, and replication (see Principle 5) by peer investigators; and ultimately to incorporate the new knowledge into the prevailing canon of the field.8
6 | A question arises as to whether this is a failure to generalize or a problem of poor implementation. The conditions under which Tennessee implemented the experiment were not reproduced in California with the now known consequence of failure to replicate and generalize. |
7 | The committee is concerned that the quality of peer review in electronic modes of dissemination varies greatly and sometimes cannot be easily assessed from its source. While the Internet is providing new and exciting ways to connect scientists and promote scientific debate, the extent to which the principles of science are met in some electronically posted work is often unclear. |
8 | Social scientists and education researchers also commonly publish information about new knowledge for practitioners and the public. In those cases, the research must be reported in accessible ways so that readers can understand the researcher’s procedures and evaluate the evidence, interpretations, and arguments. |
The goal of communicating new knowledge is self-evident: research results must be brought into the professional and public domain if they are to be understood, debated, and eventually become known to those who could fruitfully use them. The extent to which new work can be reviewed and challenged by professional peers depends critically on accurate, comprehensive, and accessible records of data, method, and inferential reasoning. This careful accounting not only makes transparent the reasoning that led to conclusions—promoting its credibility—but it also allows the community of scientists and analysts to comprehend, to replicate, and otherwise to inform theory, research, and practice in that area.
Many nonscientists who seek guidance from the research community bemoan what can easily be perceived as bickering or as an indication of “bad” science. Quite the contrary: intellectual debate at professional meetings, through research collaborations, and in other settings provide the means by which scientific knowledge is refined and accepted; scientists strive for an “open society” where criticism and unfettered debate point the way to advancement. Through scholarly critique (see, e.g., Skocpol, 1996) and debate, for example, Putnam’s work has stimulated a series of articles, commentary, and controversy in research and policy circles about the role of “social capital” in political and other social phenomena (Winter, 2000). And the Tennessee class size study has been the subject of much scholarly debate, leading to a number of follow-on analyses and launching new work that attempts to understand the process by which classroom behavior may shift in small classes to facilitate learning. However, as Lagemann (2000) has observed, for many reasons the education research community has not been nearly as critical of itself as is the case in other fields of scientific study.
The committee considered a wide range of literature and scholarship to test its ideas about the guiding principles. We realized, for example, that empiricism, while a hallmark of science, does not uniquely define it. A poet can write from first-hand experience of the world, and in this sense is an empiricist. And making observations of the world, and reasoning about their experience, helps both literary critics and historians create the
interpretive frameworks that they bring to bear in their scholarship. But empirical method in scientific inquiry has different features, like codified procedures for making observations and recognizing sources of bias associated with particular methods,9 and the data derived from these observations are used specifically as tools to support or refute knowledge claims. Finally, empiricism in science involves collective judgments based on logic, experience, and consensus.
Another hallmark of science is replication and generalization. Humanists do not seek replication, although they often attempt to create work that generalizes (say) to the “human condition.” However, they have no formal logic of generalization, unlike scientists working in some domains (e.g., statistical sampling theory). In sum, it is clear that there is no bright line that distinguishes science from nonscience or high-quality science from low-quality science. Rather, our principles can be used as general guidelines for understanding what can be considered scientific and what can be considered high-quality science (see, however, Chapters 4 and 5 for an elaboration).
To show how our principles help differentiate science from other forms of scholarship, we briefly consider two genres of education inquiry published in refereed journals and books. We do not make a judgment about the worth of either form of inquiry; although we believe strongly in the merits of scientific inquiry in education research and more generally, that “science” does not mean “good.” Rather, we use them as examples to illustrate the distinguishing character of our principles of science. The first—connoisseurship—grew out of the arts and humanities (e.g., Eisner, 1991) and does not claim to be scientific. The second—portraiture—claims to straddle the fence between humanistic and scientific inquiry (e.g., Lawrence-Lightfoot and Davis, 1997).
Eisner (1991, p. 7) built a method for education inquiry firmly rooted in the arts and humanities, arguing that “there are multiple ways in which the world can be known: Artists, writers, and dancers, as well as scientists, have important things to tell about the world.” His method of inquiry combines connoisseurship (the art of appreciation), which “aims to
9 | We do not claim that any one investigator or observational method is “objective.” Rather, the guiding principles are established to guard against bias through rigorous methods and a critical community. |
appreciate the qualities . . . that constitute an act, work, or object and, typically . . . to relate these to the contextual and antecedent conditions” (p. 85) with educational criticism (the art of disclosure), which provides “connoisseurship with a public face” (p. 85). The goal of this genre of research is to enable readers to enter an event and to participate in it. To this end, the educational critic—through educational connoisseurship— must capture the key qualities of the material, situation, and experience and express them in text (“criticism”) to make what the critic sees clear to others. “To know what schools are like, their strengths and their weaknesses, we need to be able to see what occurs in them, and we need to be able to tell others what we have seen in ways that are vivid and insightful” (Eisner, 1991, p. 23, italics in original).
Principles Of Political Science By A C Kapoor Ebook Reader Online
The grounds for his knowledge claims are not those in our guiding principles. Rather, credibility is established by: (1) structural corroboration—“multiple types of data are related to each other” (p. 110) and “disconfirming evidence and contradictory interpretations” (p. 111; italics in original) are considered; (2) consensual validation—“agreement among competent others that the description, interpretation, evaluation, and thematics of an educational situation are right” (p. 112); and (3) referential adequacy— “the extent to which a reader is able to locate in its subject matter the qualities the critic addresses and the meanings he or she ascribes to these” (p. 114). While sharing some features of our guiding principles (e.g., ruling out counterinterpretations to the favored interpretation), this humanistic approach to knowledge claims builds on a very different epistemology; the key scientific concepts of reliability, replication, and generalization, for example, are quite different. We agree with Eisner that such approaches fall outside the purview of science and conclude that our guiding principles readily distinguish them.
Portraiture (Lawrence-Lightfoot, 1994; Lawrence-Lightfoot and Davis, 1997) is a qualitative research method that aims to “record and interpret the perspectives and experience of the people they [the researchers] are studying, documenting their [the research participants’] voices and their visions—their authority, knowledge, and wisdom” (Lawrence-Lightfoot and Davis, 1997, p. xv). In contrast to connoisseurship’s humanist orientation, portraiture “seeks to join science and art” (Lawrence-Lightfoot and Davis, 1997, p. xv) by “embracing the intersection of aesthetics and empiricism” (p. 6). The standard for judging the quality of portraiture is authenticity,
“. . . capturing the essence and resonance of the actors’ experience and perspective through the details of action and thought revealed in context” (p. 12). When empirical and literary themes come together (called “resonance”) for the researcher, the actors, and the audience, “we speak of the portrait as achieving authenticity” (p. 260).
In I’ve Known Rivers, Lawrence-Lightfoot (1994) explored the life stories of six men and women:
. . . using the intensive, probing method of ‘human archeology’—a name I [Lawrence-Lightfoot] coined for this genre of portraiture as a way of trying to convey the depth and penetration of the inquiry, the richness of the layers of human experience, the search for ancestral and generational artifacts, and the painstaking, careful labor that the metaphorical dig requires. As I listen to the life stories of these individuals and participate in the ‘co-construction’ of narrative, I employ the themes, goals, and techniques of portraiture. It is an eclectic, interdisciplinary approach, shaped by the lenses of history, anthropology, psychology and sociology. I blend the curiosity and detective work of a biographer, the literary aesthetic of a novelist, and the systematic scrutiny of a researcher (p. 15).
Some scholars, then, deem portraiture as “scientific” because it relies on the use of social science theory and a form of empiricism (e.g., interview). While both empiricism and theory are important elements of our guiding principles, as we discuss above, they are not, in themselves, defining. The devil is in the details. For example, independent replication is an important principle in our framework but is absent in portraiture in which researcher and subject jointly construct a narrative. Moreover, even when our principles are manifest, the specific form and mode of application can make a big difference. For example, generalization in our principles is different from generalization in portraiture. As Lawrence-Lightfoot and Davis (1997) point out, generalization as used in the social sciences does not fit portraiture. Generalization in portraiture “. . . is not the classical conception . . . where the investigator uses codified methods for generalizing from specific findings to a universe, and where there is little interest in findings that reflect only the characteristics of the sample. . . .” By contrast, the portraitist seeks to “document and illuminate the complexity
and detail of a unique experience or place, hoping the audience will see itself reflected in it, trusting that the readers will feel identified. The portraitist is very interested in the single case because she believes that embedded in it the reader will discover resonant universal themes” (p. 15). We conclude that our guiding principles would distinguish portraiture from what we mean by scientific inquiry, although it, like connoisseurship, has some traits in common.
To this point, we have shown how our principles help to distinguish science and nonscience. A large amount of education research attempts to base knowledge claims on science; clearly, however, there is great variation with respect to scientific rigor and competence. Here we use two studies to illustrate how our principles demonstrate this gradation in scientific quality.
The first study (Carr, Levin, McConnachie, Carlson, Kemp, Smith, and McLaughlin, 1999) reported on an educational intervention carried out on three nonrandomly selected individuals who were suffering severe behavioral disorders and who were residing in group-home settings. Since earlier work had established remedial procedures involving “simulations and analogs of the natural environment” (p. 6), the focus of the study was on the generalizability (or external validity) to the “real world” of the intervention (places, caregivers).
Over a two to three week period, “baseline” frequencies of their problem behaviors were established, these behaviors were remeasured after an intervention lasting for some years was carried out. The researchers took a third measurement during the maintenance phase of the study. While care was taken in describing behavioral observations, variable construction and reliability, the paper reporting on the study did not provide clear, detailed depictions of the interventions or who carried them out (research staff or staff of the group homes). Furthermore, no details were given of the changes in staffing or in the regimens of the residential settings—changes that were inevitable over a period of many years (the timeline itself was not clearly described). Finally, in the course of daily life over a number of years, many things would have happened to each of the subjects, some of which might be expected to be of significance to the study, but none of them were documented. Over the years, too, one might expect some developmental changes to occur in the aggressive behavior displayed by the research subjects, especially in the two teenagers. In short, the study focused on
generalizability at too great an expense relative to internal validity. In the end, there were many threats to internal validity in this study, and so it is impossible to conclude (as the authors did) from the published report that the “treatment” had actually caused the improvement in behavior that was noted.
Turning to a line of work that we regard as scientifically more successful, in a series of four randomized experiments, Baumeister, Bratslavsky, Muraven, and Tice (1998) tested three competing theories of “will power” (or, more technically, “self-regulation”)—the psychological characteristic that is posited to be related to persistence with difficult tasks such as studying or working on homework assignments. One hypothesis was that will power is a developed skill that would remain roughly constant across repeated trials. The second theory posited a self-control schema “that makes use of information about how to alter one’s own response” (p. 1254) so that once activated on one trial, it would be expected to increase will power on a second trial. The third theory, anticipated by Freud’s notion of the ego exerting energy to control the id and superego, posits that will power is a depletable resource—it requires the use of “psychic energy” so that performance from trial 1 to trial 2 would decrease if a great deal of will power was called for on trial 1. In one experiment, 67 introductory psychology students were randomly assigned to a condition in which either no food was present or both radishes and freshly baked chocolate chip cookies were present, and the participants were instructed either to eat two or three radishes (resisting the cookies) or two or three cookies (resisting the radishes). Immediately following this situation, all participants were asked to work on two puzzles that unbeknownst to them, were unsolvable, and their persistence (time) in working on the puzzles was measured. The experimental manipulation was checked for every individual participating by researchers observing their behavior through a one-way window. The researchers found that puzzle persistence was the same in the control and cookie conditions and about 2.5 times as long, on average, as in the radish condition, lending support to the psychic energy theory—arguably, resisting the temptation to eat the cookies evidently had depleted the reserve of self-control, leading to poor performance on the second task. Later experiments extended the findings supporting the energy theory to situations involving choice, maladaptive performance, and decision making.
However, as we have said, no single study or series of studies satisfy all of our guiding principles, and these will power experiments are no exception. They all employed small samples of participants, all drawn from a college population. The experiments were contrived—the conditions of the study would be unlikely outside a psychology laboratory. And the question of whether these findings would generalize to more realistic (e.g., school) settings was not addressed.
Principles Of Political Science By A C Kapoor Ebook Reader Pdf
Nevertheless, the contrast in quality between the two studies, when observed through the lens of our guiding principles, is stark. Unlike the first study, the second study was grounded in theory and identified three competing answers to the question of self-regulation, each leading to a different empirically refutable claim. In doing so, the chain of reasoning was made transparent. The second study, unlike the first, used randomized experiments to address counterclaims to the inference of psychic energy, such as selectivity bias or different history during experimental sessions. Finally, in the second study, the series of experiments replicated and extended the effects hypothesized by the energy theory.
Nearly a century ago, John Dewey (1916) captured the essence of the account of science we have developed in this chapter and expressed a hopefulness for the promise of science we similarly embrace:
Our predilection for premature acceptance and assertion, our aversion to suspended judgment, are signs that we tend naturally to cut short the process of testing. We are satisfied with superficial and immediate short-visioned applications. If these work out with moderate satisfactoriness, we are content to suppose that our assumptions have been confirmed. Even in the case of failure, we are inclined to put the blame not on the inadequacy and incorrectness of our data and thoughts, but upon our hard luck and the hostility of circumstances. . . . Science represents the safeguard of the [human] race against these natural propensities and the evils which flow from them. It consists of the special appliances and methods.. slowly worked out in order to conduct reflection under conditions whereby its procedures and results are tested.